forward: https://alpopkes.com/posts/python/packaging_tools/
Last update
This post was last updated on August 29th, 2024.
Motivation
When I started with Python and created my first package I was confused. Creating and managing a package seemed much harder than I expected. In addition, multiple tools existed and I wasn’t sure which one to use. I’m sure most of you had the very same problem in the past. Python has a zillion tools to manage virtual environments and create packages and it can be hard (or almost impossible) to understand which one fits your needs. Several talks and blog post on the topic exist, but none of them gives a complete overview or evaluates the tools in a structured fashion. This is what this post is about. I want to give you a truly unbiased evaluation of existing packaging and environment management tools. In case you’d rather watch a talk, take a look at the recording of PyCon DE 2023 or EuroPython 2023.
Categorization
For the purpose of this article I identified five main categories that are important when it comes to environment and package management:
- Environment management (which is mostly concerned with virtual environments)
- Package management
- Python version management
- Package building
- Package publishing
As you can see in the Venn diagram below, lots of tools exist. Some can do a single thing (i.e. they are single-purpose), others can perform multiple tasks (hence I call them multi-purpose tools).
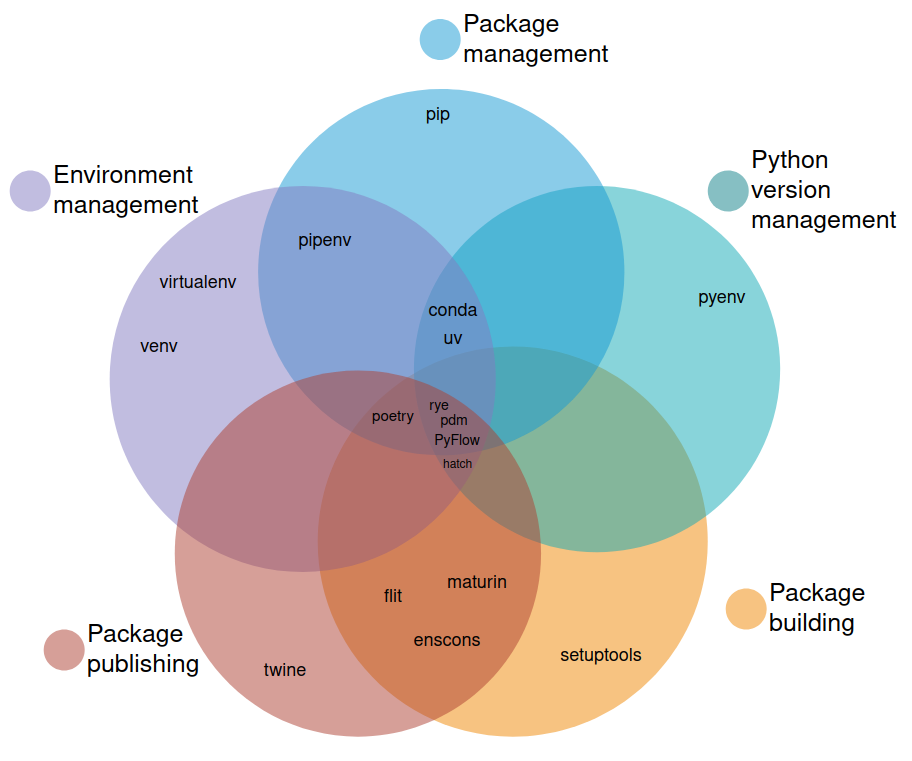
Let’s walk through the categories keeping a developers perspective in mind. Let’s say you are working on a personal project alongside your work projects. At work you’re using Python 3.7 whereas your personal project should be using the newest Python version (currently 3.11). In other words: you want to be able to install different Python versions and switch between them. That’s what our first category, Python version management is about.
Within your projects you are using other packages (e.g. pandas
or sklearn
for data science). These are dependencies of your project that you have
to install and manage (e.g. upgrade when new versions are released).
This is what package management is about.
Because
different projects might require different versions of the same package
you need to create (and manage) virtual environments to avoid dependency
conflicts. Tools for this are collected in the category environment management. Most tools use virtual environments, but some use another concept called “local packages” which we will look at later.
Once
your code is in a proper state you might want to share it with fellow
developers. For this you first have to build your package (package building) before you can publish it to PyPI or another index (package publishing).
In the following we will look at each of the categories in more detail, including a short definition, motivation and the available tools. I will present some single-purpose tools in more detail and several multi-purpose tools in a separate section at the end. Let’s get started with the first category: Python version management.
Python version management
Definition
A tool that can perform Python version management allows you to install Python versions and switch between them easily.
Motivation
Why would we want to use different Python versions? There are several reasons. For example, you might be working of several projects where each projects requires a different Python version. Or you might develop a project that supports several Python versions and you want to test all of them. Besides that it can be nice to check out what the newest Python version has to offer, or test a pre-release version of Python for bugs.
Tools
Our Venn diagram displays the available tools for Python version management: pyenv, conda, rye, pdm, hatch
and PyFlow
. We will first look at pyenv
and consider the multi-purpose tools in a separate section.
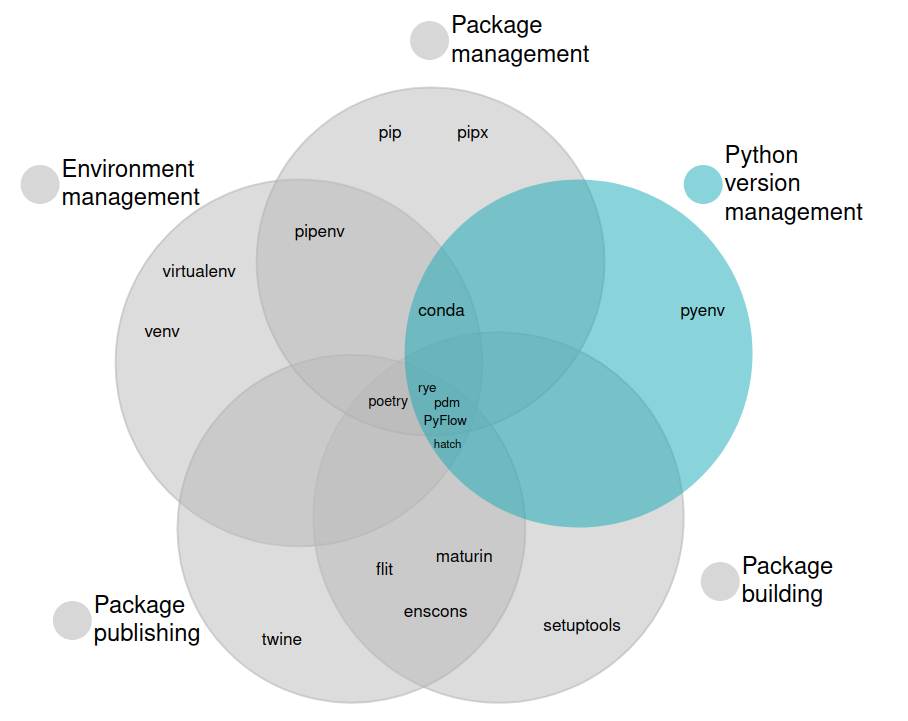
pyenv
Python has one single-purpose tool that lets you install and manage Python versions: pyenv! Pyenv is easy to use. The most important commands are the following:
# Install specific Python version
pyenv install 3.10.4
# Switch between Python versions
pyenv shell <version> # select version just for current shell session
pyenv local <version> # automatically select version whenever you are in the current directory
pyenv global <version> # select version globally for your user account
(Virtual) environment management
Definition
A tool that can perform environment management allows you to create and manage (virtual) environments.
Motivation
Why do we want to use environments in the first place? As mentioned in the beginning, projects have specific requirements (i.e. they depend on other packages). It’s often the case that different projects require different versions of the same package. This can cause dependency conflicts. In addition, problems can occur when using pip install
to install a package because the package is placed with your system-wide Python installation. Some of these problems can be solved by using the --user
flag in the pip
command. However, this option might not be known to everyone, especially beginners.
Tools
Many tools allow users to create and manage environments. These are: venv, virtualenv, pipenv, conda, pdm, poetry, hatch, rye
and PyFlow
. Only two of them are single-purpose tools: venv
and virtualenv
. Let’s look at both of them in more detail.
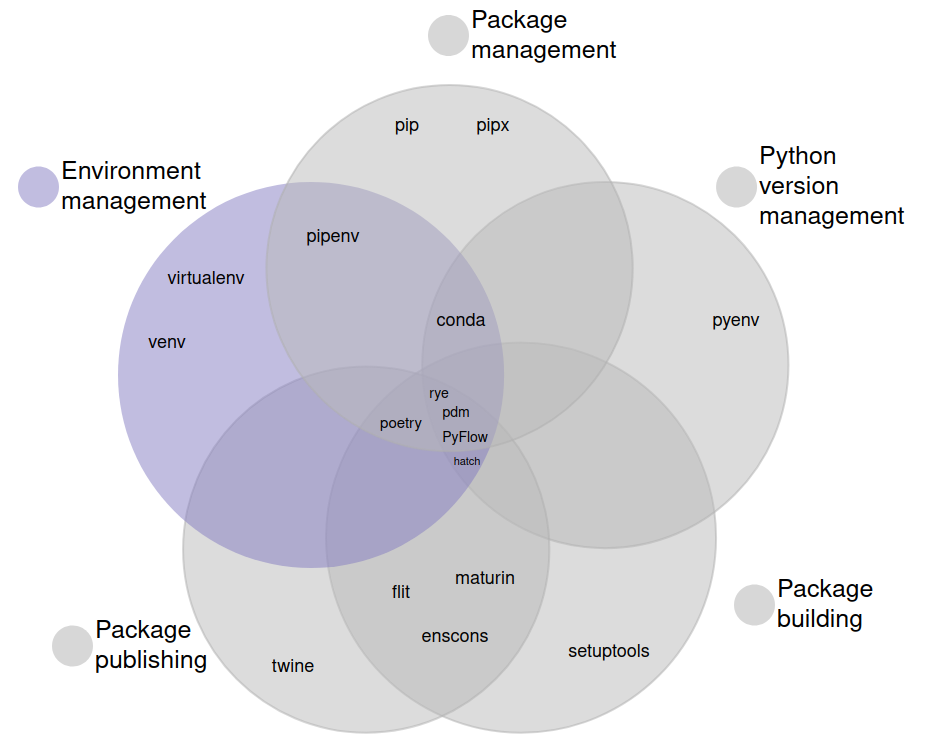
venv
Venv is the built-in Python package for creating virtual environments. This means that it is shipped with Python and does not have to be installed by the user. The most important commands are the following:
# Create new environment
python3 -m venv <env_name>
# Activate an environment
. <env_name>/bin/activate
# Deactivate an active environment
deactivate
virtualenv
Virtualenv tries to improve venv
. It offers more features than venv
and is faster and more powerful. The most important commands are similar to the ones of venv
, only creating a new environment is cleaner:
# Create new environment
virtualenv <env_name>
# Activate an environment
. <env_name>/bin/activate
# Deactivate an active environment
deactivate
Recap I - pyproject.toml
Before we can talk about packaging I want to make sure that you are aware of the most important file for packaging: pyproject.toml
.
Packaging in Python has come a long way. Until PEP 518 setup.py
files where used for packaging, using setuptools
as a build tool. PEP 518 introduced the usage of a pyproject.toml
file. As a consequence, you always need a pyproject.toml
file when creating a package. pyproject.toml
is used to define the settings of a project, define metadata and lots of other things. If you would like to see an example check out the pyproject.toml
file of the pandas library. With the knowledge on pyproject.toml
we can go on at take a look at package management.
Package management
Definition
A tool that can perform package management is able to download and install libraries and their dependencies.
Motivation
Why do we care about packages? Packages allow us to define a hierarchy of modules and to access modules easily using the dot-syntax (from package.module import my_function
). In addition, they make it easy to share code with other developers. Since each package contains a pyproject.toml
file which defines its dependencies, other developers don’t have to install the required packages separately but can simply install the package from its pyproject.toml
file.
Tools
Lots of tools can perform package management: pip, pipx, pipenv, conda, pdm, poetry, rye
and PyFlow
. The single-purpose tool for package management is pip
which is well known in the Python community.
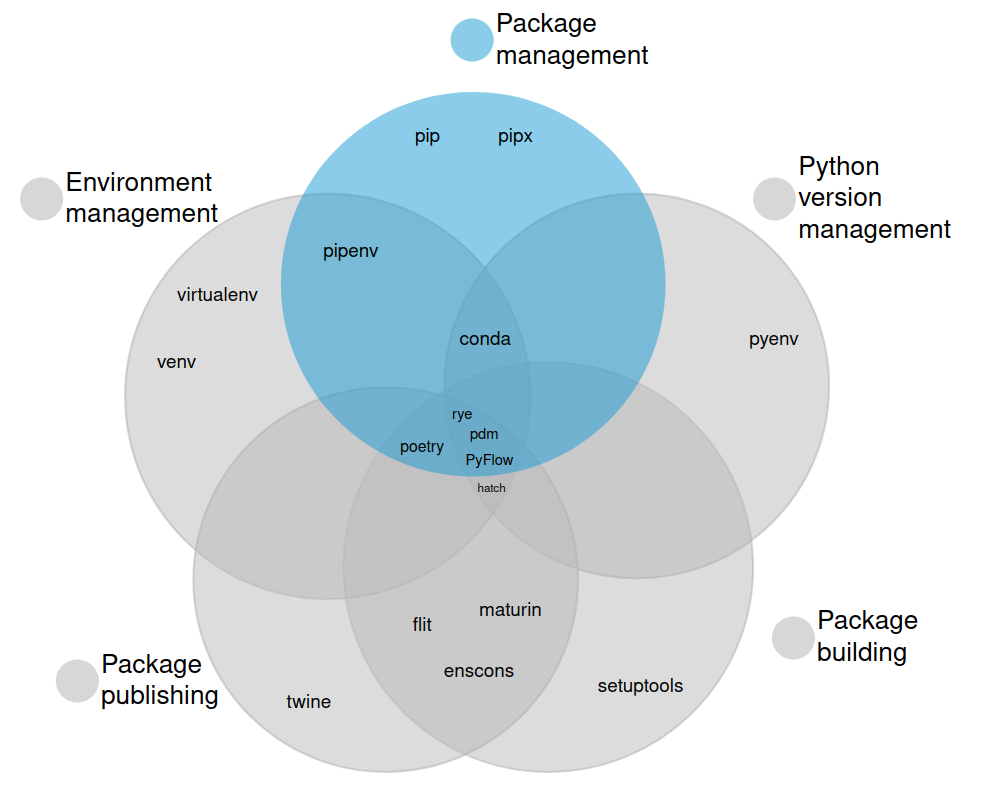
pip
The standard package manager for Python is pip
. It’s shipped with Python and allows you to install packages from PyPI and other indexes. The main command (probably one of the first commands a Python developer learns) is pip install <package_name>
. Of course, pip
offers lots of other options. Check out the documentation for more information about available flags, etc.
Recap II - Lock file
Before we go on to the multi-purpose tools, there is one more file that’s important for packaging: the lock file. While pyproject.toml
contains abstract dependencies, a lock file contains concrete dependencies. It records exact versions of all dependencies installed for a project (e.g. pandas==2.0.3
). This enables reproducibility of projects across multiple platforms. If you have never seen a lock file before, take a look at this one from poetry
:
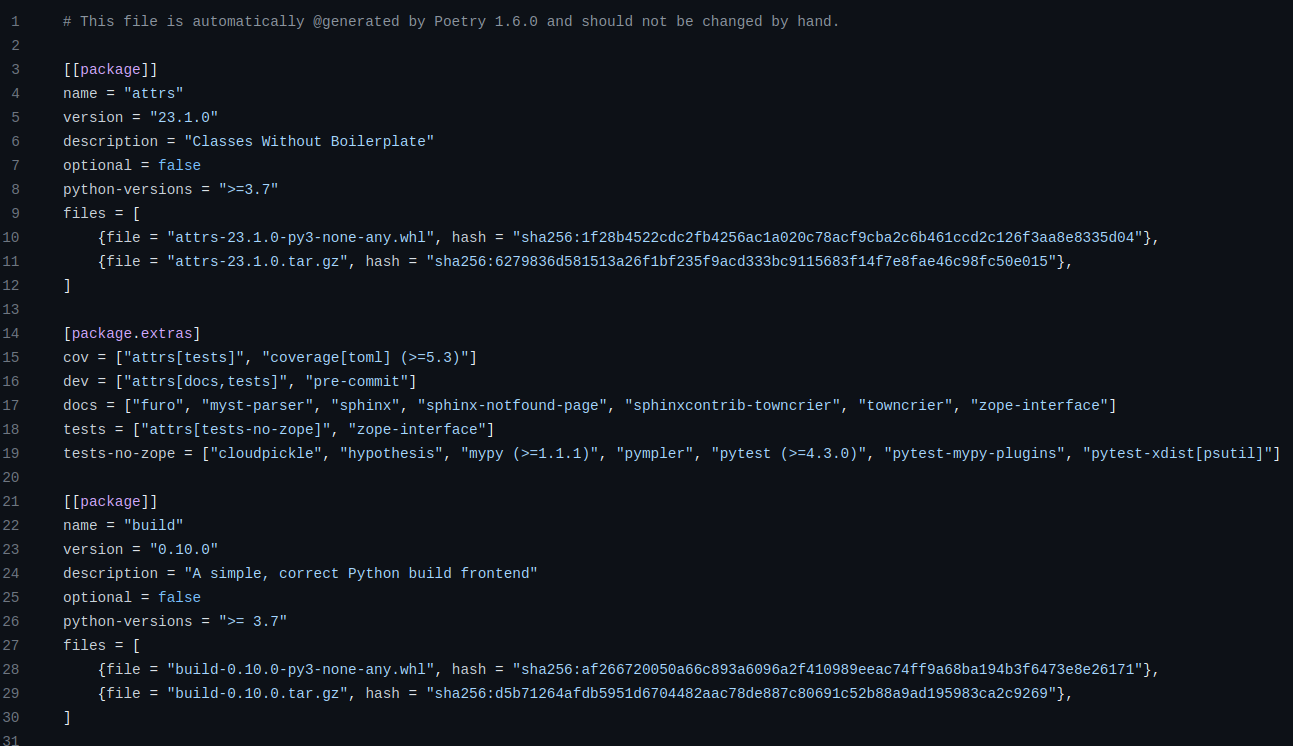
Multi-purpose tools
Knowing about lock files we can start looking at tools that perform several tasks. We will start with pipenv
and conda
before we transition to packaging tools like poetry
and pdm
.
Pipenv
As the name suggests, pipenv
combines pip
and virtualenv
. It allows you to perform virtual environment management and package management as we can see in our Venn diagram:
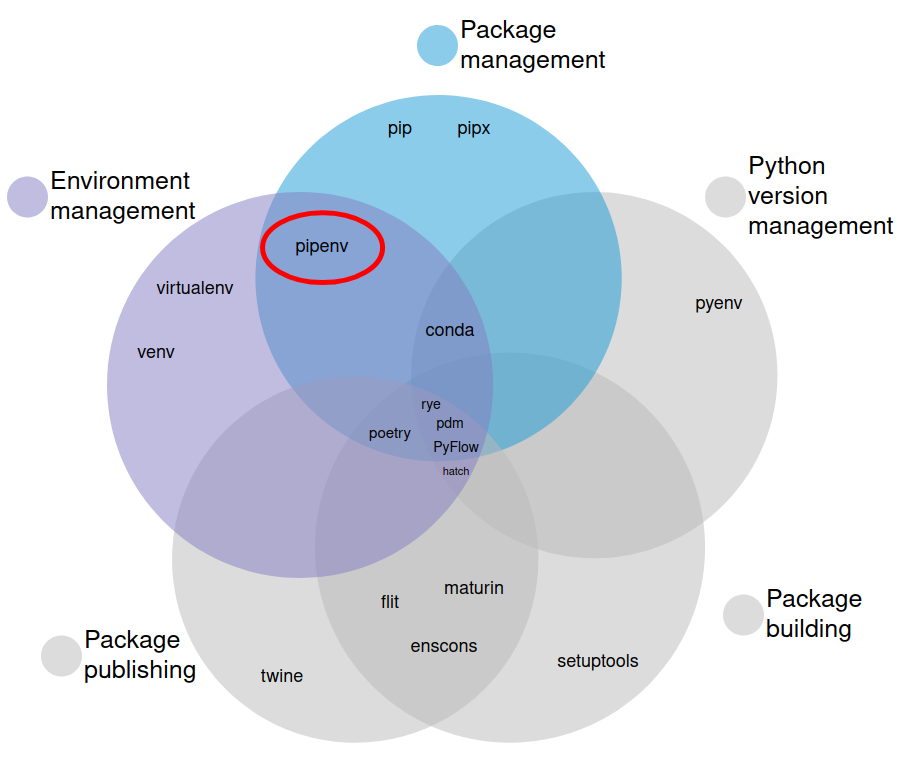
pipenv
introduces two additional files:
Pipfile
Pipfile.lock
Pipfile
is a TOML file (similar to pyproject.toml
) used to define project dependencies. It is managed by the developer when she invokes pipenv
commands (like pipenv install
). Pipfile.lock
allows for deterministic builds. It eliminates the need for a requirements.txt
file and is managed automatically through locking actions .
The most important pipenv
commands are:
# Install package
pipenv install <package_name>
# Run Python script within virtual env
pipenv run <script_name.py>
# Activate virtual env
pipenv shell
Conda
Conda
is a general-purpose package management system. That means that it’s not limited to Python packages. Conda is a huge tool with lots of capabilities. Lot’s of tutorials and blog posts exist (for example the official one) so I won’t go into more detail here. However, I want to mention one thing: while it is possible to build and publish a package with conda
I did not include the tool in the appropriate categories. That’s because packaging with conda
works a little differently and the resulting packages will be conda
packages.
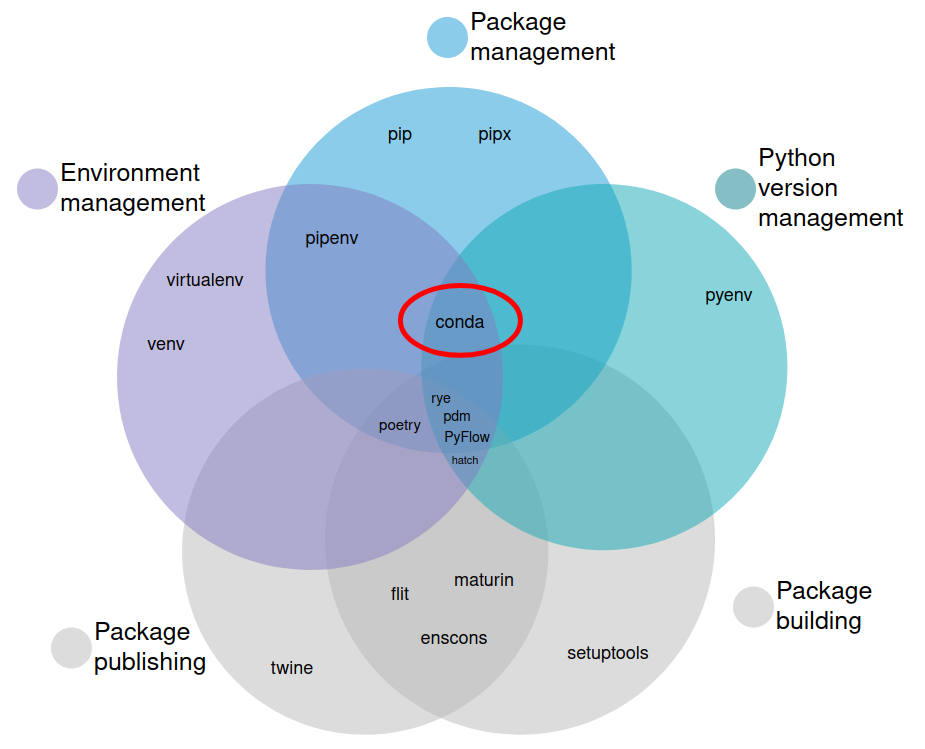
Feature evaluation
Last but not least I want to present multi-purpose tools for packaging. I promised an unbiased evaluation. For this purpose I created a list of features that I consider important when comparing different tools. The features are:
Does the tool manage dependencies? | ? |
Does it resolve/lock dependencies? | ? |
Is there a clean build/publish flow? | ? |
Does it allow to use plugins? | ? |
Does it support PEP 660 (editable installs)? | ? |
Does it support PEP 621 (project metadata)? | ? |
Regarding the two PEPs: Python has a lot of open and closed PEPs on packaging. For a full overview take a look at this page. I only included PEP 660 and PEP 621 for specific reasons:
- PEP 660 is about editable installs for
pyproject.toml
based builds. When you install a package usingpip
you have the option to install it in editable mode usingpip install -e package_name
. This is an important features to have when you are developing a package and want your changes to be directly reflected in your environment. - PEP 621 specifies how to write a project’s core metadata in a
pyproject.toml
file. I added it because one package (spoiler: it’spoetry
) currently does not support this PEP but uses its own way for declaring metadata.
Flit
Flit tries to create a simple way to put Python packages and modules on PyPI. It has a very specific use case: it’s meant to be used for packaging pure Python packages (that is, packages without a build step). It doesn’t care about any of the other tasks:
- Python version management: ❌
- Package management: ❌
- Environment management: ❌
- Building a package: ✅
- Publishing a package: ✅
This is also reflected in our Venn diagram:
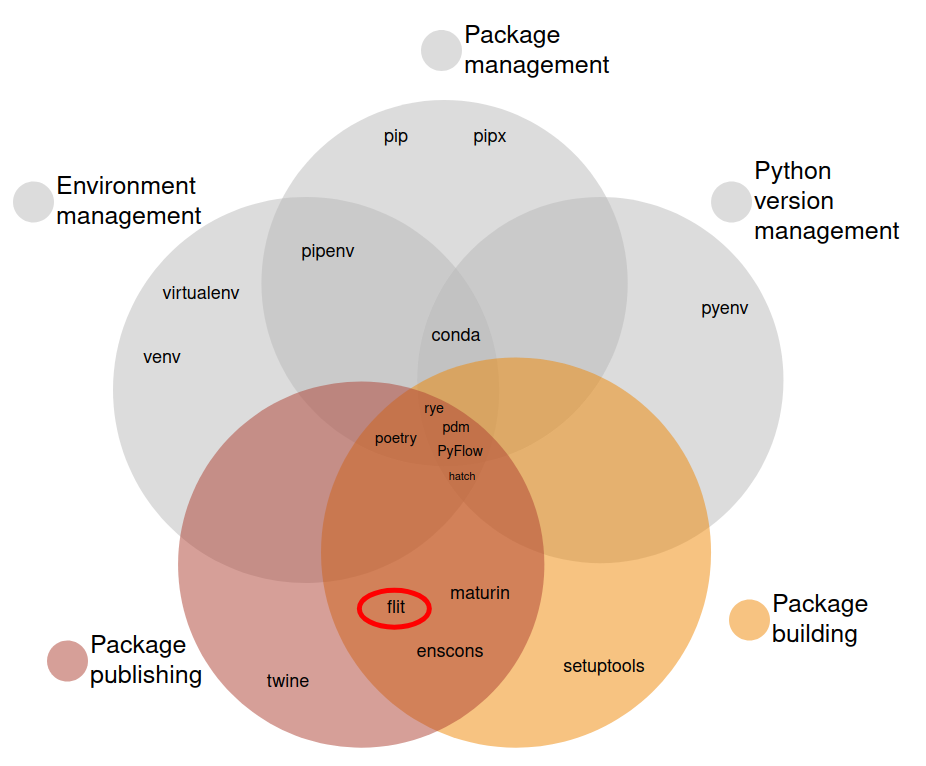
Feature evaluation
Does the tool manage dependencies? | ❌ |
Does it resolve/lock dependencies? | ❌ |
Is there a clean build/publish flow? | ✅ |
Does it allow to use plugins? | ❌ |
Does it support PEP 660 (editable installs)? | ✅ |
Does it support PEP 621 (project metadata)? | ✅ |
Main commands
# Create new pyproject.toml
flit init
# Build and publish
flit publish
Poetry
Poetry is a well known tool in the packaging world. As visible in the Venn diagram it can do everything except for Python version management:
- Python version management: ❌
- Package management: ✅
- Environment management: ✅
- Building a package: ✅
- Publishing a package: ✅
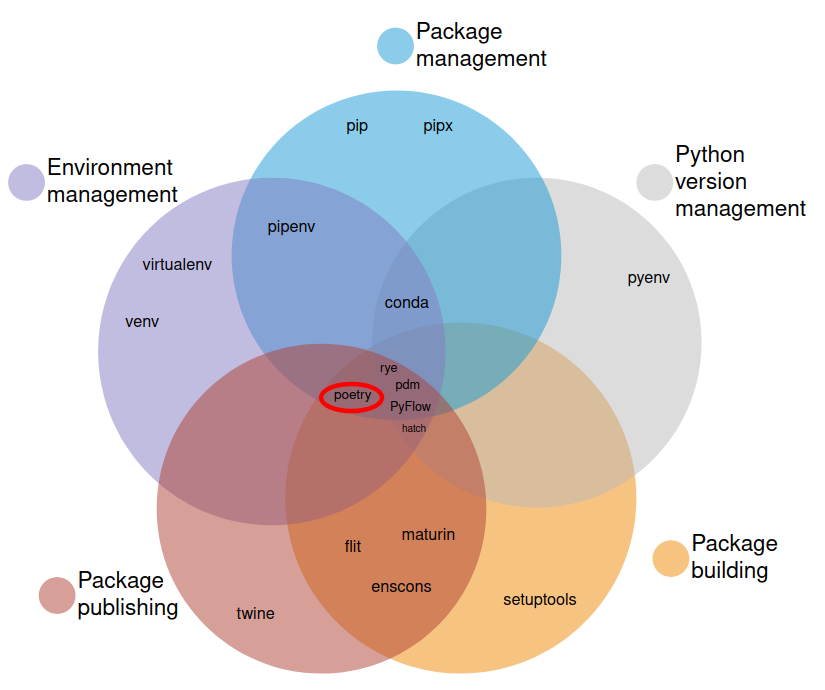
Taking a look at the feature evaluation below you will see than Poetry does not support PEP 621. There has been an open issue about this on GitHub for about 1.5 years, but it hasn’t been integrated into the main code base (yet).
Update (29.08.2024): The next major release of Poetry (Poetry 2.0) will support PEP 621 (see this discussion on GitHub).
Feature evaluation
Does the tool manage dependencies? | ✅ |
Does it resolve/lock dependencies? | ✅ |
Is there a clean build/publish flow? | ✅ |
Does it allow to use plugins? | ✅ |
Does it support PEP 660 (editable installs)? | ✅ |
Does it support PEP 621 (project metadata)? | ❌ |
Main commands
# Create directory structure and pyproject.toml
poetry new <project_name>
# Create pyproject.toml interactively
poetry init
# Install package from pyproject.toml
poetry install
Dependency management
# Add dependency
poetry add <package_name>
# Display all dependencies
poetry show --tree
Running code
# Activate virtual env
poetry shell
# Run script within virtual env
poetry run python <script_name.py>
Lock file
When installing a package for the first time, Poetry resolves all dependencies listed in your pyproject.toml
file and downloads the latest version of the packages. Once Poetry has finished installing, it writes all the packages and the exact versions that it downloaded to a poetry.lock
file, locking the project to those specific versions. It’s recommended to commit the lock file to your project repo so that all people working on the project are locked to the same versions of dependencies. To update your dependencies to the latest versions, use the poetry update
command.
Build/publish flow
# Package code (creates `.tar.gz` and `.whl` files)
poetry build
# Publish to PyPI
poetry publish
PDM
PDM is a relatively new package and dependency manager (started in 2019) that is strongly inspired by Poetry and PyFlow. You will notice that I’m not talking about PyFlow in this article. That’s because PyFlow is not actively developed anymore - a must in the quickly evolving landscape of packaging. Being a new(er) tool, PDM requires Python 3.7 or higher. Another difference to other tools is that PDM allows users to choose a build backend.
PDM is the only tool (apart from PyFlow) that implements PEP 582 on local packages, an alternative way of implementing environment management. Note that this PEP was recently rejected.
As visible in the Venn diagram, PDM sits right next to Poetry. That means that it can do everything except for Python version management.
Update (29.08.2024): PDM has added the functionality to install Python versions using the pdm python install
command (official documentation). You can switch between different Python versions using the pdm use
command (official documentation).
The updated table now looks as follows:
- Python version management: ✅
- Package management: ✅
- Environment management: ✅
- Building a package: ✅
- Publishing a package: ✅
The main commands of PDM are similar to Poetry. However, less commands exist. For example, there is no pdm shell
or pdm new
at the moment.
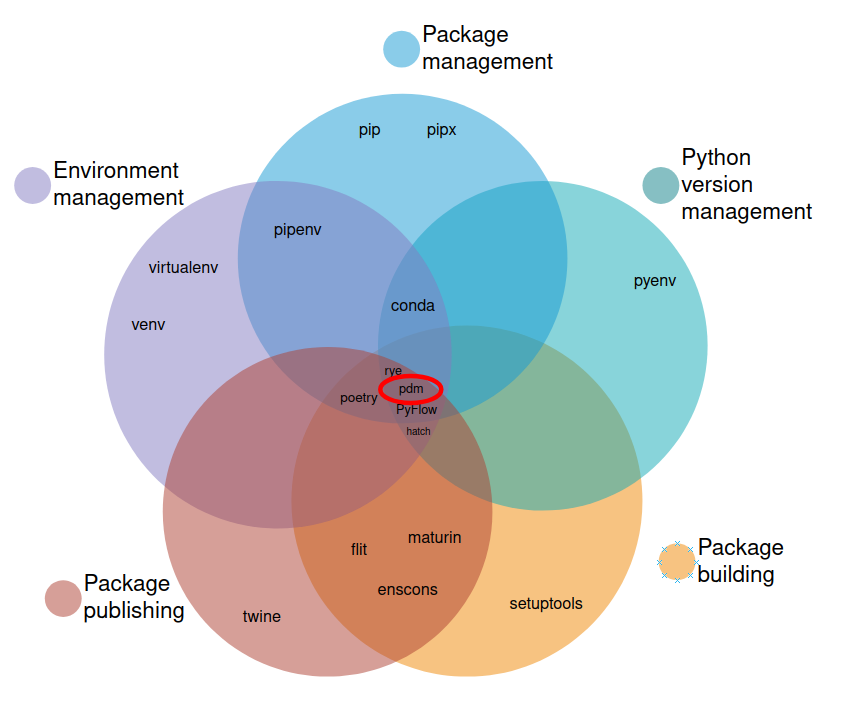
Feature evaluation
Does the tool manage dependencies? | ✅ |
Does it resolve/lock dependencies? | ✅ |
Is there a clean build/publish flow? | ✅ |
Does it allow to use plugins? | ✅ |
Does it support PEP 660 (editable installs)? | ✅ |
Does it support PEP 621 (project metadata)? | ✅ |
Creating a new project
# Create pyproject.toml interactively
pdm init
# Install package from pyproject.toml
pdm install
Dependency management
# Add dependency
pdm add <package_name>
# Display all dependencies
pdm list --graph
Running code
# No pdm shell command
# Run script within env
pdm run python <script_name.py>
Lock file
The locking functionality of PDM is similar to Poetry. When installing a package for the first time, PDM resolves all dependencies listed in your pyproject.toml
file and downloads the latest version of the packages. Once PDM has finished installing, it writes all packages and the exact versions that it downloaded to a pdm.lock
file, locking the project to those specific versions. It’s recommended to commit the lock file to your project repo so that all people working on the project are locked to the same versions of dependencies. To update your dependencies to the latest versions, use the pdm update
command.
Build/publish flow
# Package code (creates `.tar.gz` and `.whl` files)
pdm build
# Publish to PyPI
pdm publish
Hatch
Hatch can perform the following tasks:
- Python version management: ✅
- Package management: ❌
- Environment management: ✅
- Building a package: ✅
- Publishing a package: ✅
Update: Since version 1.8.0, Hatch provides the ability to manage Python installations, e.g. using hatch python install
. Currently, only major.minor versions can be installed like 3.7 or 3.8, but not specific patches like 3.7.4.
It should be noted that the author of Hatch promised that locking functionality will be added soon, which should also enable package management. Please make sure to check the latest version of Hatch to see if this has been implemented when you read this article.
Update (29.08.2024): Hatch still does not have locking functionality and is hence not able to perform package management.
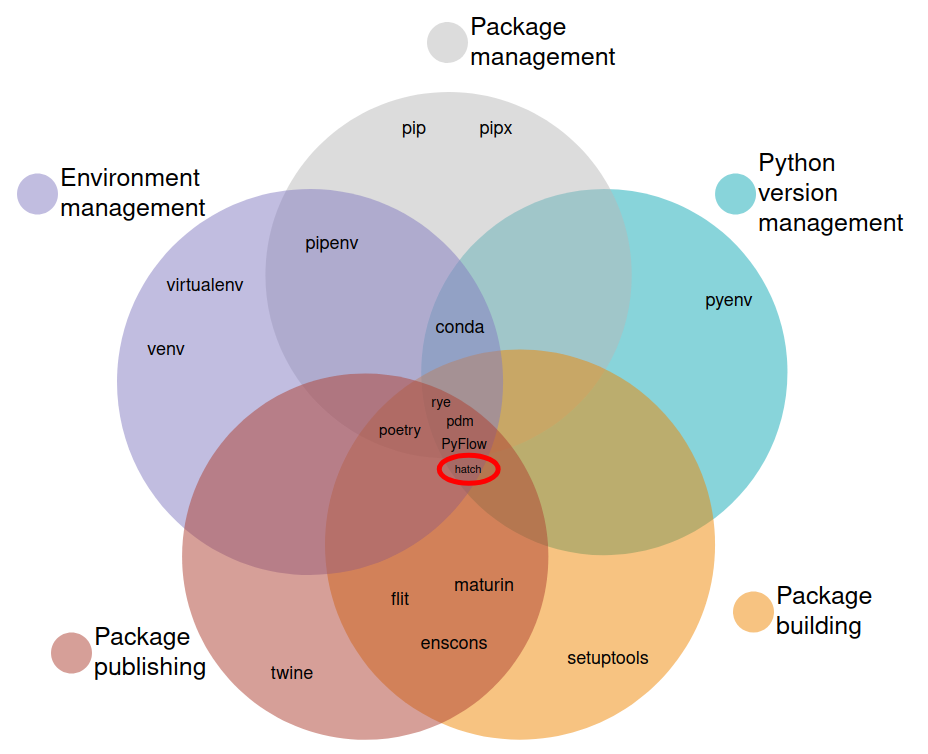
Feature evaluation
Does the tool manage dependencies? | ❌ |
Does it resolve/lock dependencies? | ❌ |
Is there a clean build/publish flow? | ✅ |
Does it allow to use plugins? | ✅ |
Does it support PEP 660 (editable installs)? | ✅ |
Does it support PEP 621 (project metadata)? | ✅ |
Creating a new project
# Create directory structure and pyproject.toml
hatch new <project_name>
# Interactive mode
hatch new -i <project_name>
# Initialize existing project / create pyproject.toml
hatch new --init
Dependency management
# Packages are added manually to pyproject.toml
hatch add <package_name> # This command doesn't exist!
# Display dependencies
hatch dep show table
Running code
# Activate virtual env
hatch shell
# Run script within virtual env
hatch run python <script_name.py>
Build/publish flow
# Package code (creates `.tar.gz` and `.whl` files)
hatch build
# Publish to PyPI
hatch publish
Declarative environment management
Special about Hatch is that it allows you to configure your virtual environments within the pyproject.toml
file. In addition it lets you define scripts specifically for an environment. And example use case for this is code formatting.
Rye
Rye was recently developed by Armin Ronacher (first release May 2023), the creator of the Flask framework. It is strongly inspired by rustup
and cargo
, the packaging tools of the programming language Rust. Rye is written in Rust and is able to perform all tasks in our Venn diagram:
- Python version management: ✅
- Package management: ✅
- Environment management: ✅
- Building a package: ✅
- Publishing a package: ✅
Currently, Rye does not have a plugin interface. However, since new releases are published on a regular basis, this might be added in the future.
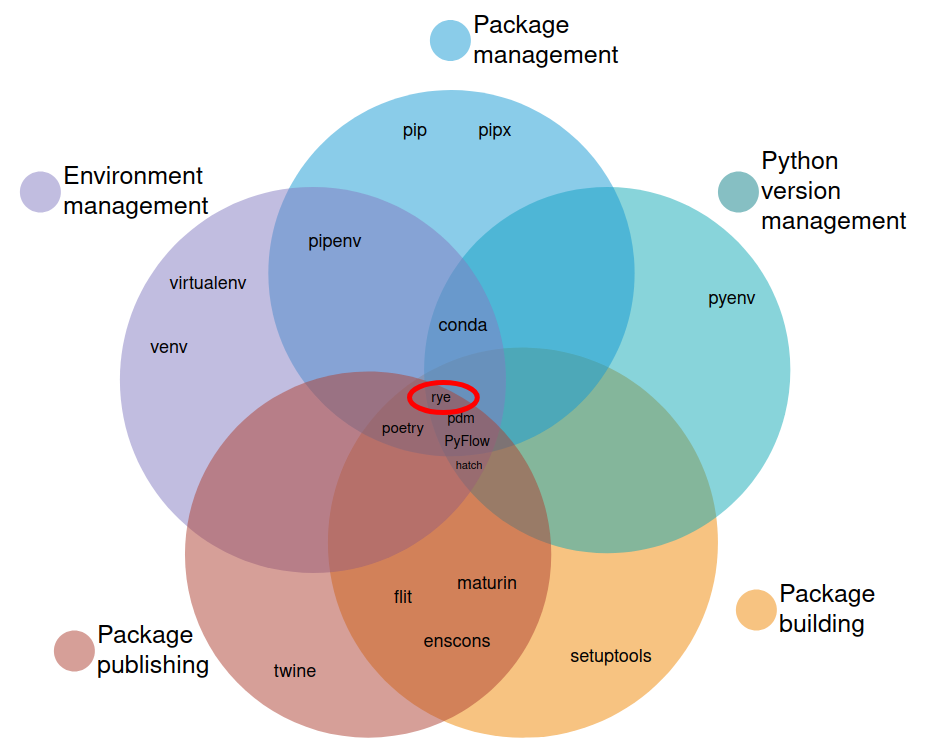
Feature evaluation
Does the tool manage dependencies? | ✅ |
Does it resolve/lock dependencies? | ✅ |
Is there a clean build/publish flow? | ✅ |
Does it allow to use plugins? | ❌ |
Does it support PEP 660 (editable installs)? | ✅ |
Does it support PEP 621 (project metadata)? | ✅ |
Creating a new project
# Create directory structure and pyproject.toml
rye init <project_name>
# Pin a Python version
rye pin 3.10
Dependency management
# Add dependency - this does not install the package!
rye add <package_name>
# Synchronize virtual envs, lock file, etc.
# This install packages and Python versions
rye sync
Running code
# Activate virtual env
rye shell
# Run script within virtual env
rye run python <script_name.py>
Build/publish flow
# Package code (creates `.tar.gz` and `.whl` files)
rye build
# Publish to PyPI
rye publish
uv
The newes addition to the Python packaging landscape is uv
. Similar to rye
, uv
is written in Rust. Actually, the company behind uv
(Astral) has taken stewardship of rye
and will “maintain Rye as we expand uv into a unified successor project”. At the moment, uv
can do everything besides building and publishing a package. However, this might change in the future. Therefore, I haven’t added uv
to all Venn diagrams yet. To dive deeper into uv
, check out the official documentation.
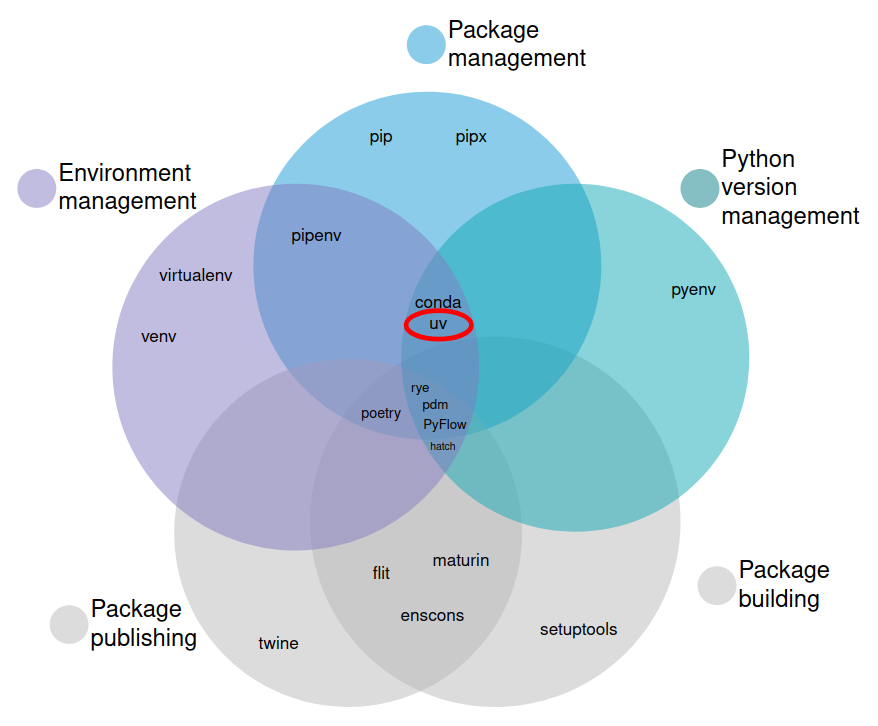
- Python version management: ✅
- Package management: ✅
- Environment management: ✅
- Building a package: ❌
- Publishing a package: ❌
Creating a new project
# Create directory structure and pyproject.toml
uv init <project_name>
# Install a specific Python version
uv python install 3.12.3
Dependency management
# Add dependency
uv add <package_name>
# Remove a dependene
uv remove <package_name>
Running code
# Run script within virtual env
uv run <script_name.py>
# Alternatively, you can manually update the environment
# and then activate it before executing a command
uv sync
source .venv/bin/activate
python <script_name.py>
Overview
Flit | Poetry | PDM | Hatch | Rye | uv | |
---|---|---|---|---|---|---|
Does the tool manage dependencies? | ❌ | ✅ | ✅ | ❌ | ✅ | ✅ |
Does it resolve/lock dependencies? | ❌ | ✅ | ✅ | ❌ | ✅ | ✅ |
Is there a clean build/publish flow? | ✅ | ✅ | ✅ | ✅ | ✅ | ❌ |
Does it allow to use plugins? | ❌ | ✅ | ✅ | ✅ | ❌ | ❌ |
Does it support PEP 660 (editable installs)? | ✅ | ✅ | ✅ | ✅ | ✅ | ✅ |
Does it support PEP 621 (project metadata)? | ✅ | ❌ | ✅ | ✅ | ✅ | ✅ |
Tools that do not fit the categories
Some tools exist which don’t fit into any of my categories. These are:
- pip-tools which helps to keep the versions of your
pip
-based packages up-to-date. - tox and nox which are mainly used for testing but also handles virtual environments.