onnx导出-多输入+动态维度
目录常见问题
多参数输入
import numpy as np
import cv2
import torch
import torch.nn as nn
import torchvision
import onnx
import onnxruntime
print(torch.__version__)
print(torchvision.__version__)
# 1.13.0+cu116
# 0.14.0+cu116
class SRNNnet(nn.Module):
def __init__(self, upscale_factor=3):
super().__init__()
self.upscale_factor = upscale_factor
self.img_upsampler = nn.Upsample(
scale_factor=self.upscale_factor,
mode='bicubic',
align_corners=False)
self.conv1 = nn.Conv2d(3,64,kernel_size=9,padding=4)
self.conv2 = nn.Conv2d(64,32,kernel_size=1,padding=0)
self.conv3 = nn.Conv2d(32,3,kernel_size=5,padding=2)
self.relu = nn.ReLU()
def forward(self, x):
x = self.img_upsampler(x)
out = self.relu(self.conv1(x))
out = self.relu(self.conv2(out))
out = self.conv3(out)
return out
def print_state_dict(state_dict):
print(len(state_dict))
for layer in state_dict:
print(layer, '\t', state_dict[layer].shape)
def init_torch_model():
torch_model = SRNNnet(upscale_factor=3)
state_dict = torch.load('assets/srcnn.pth')['state_dict']
print_state_dict(state_dict)
# Adapt the checkpoint
for old_key in list(state_dict.keys()):
new_key = '.'.join(old_key.split('.')[1:])
state_dict[new_key] = state_dict.pop(old_key)
torch_model.load_state_dict(state_dict)
torch_model.eval()
print("init_torch_model success")
return torch_model
def test_mode():
torch_model = init_torch_model()
input_img = cv2.imread('assets/dog.jpg').astype(np.float32)
# # 固定图像大小为256x256
input_img = cv2.resize(input_img,(256,256))
# HWC to NCHW
input_img = np.transpose(input_img, [2, 0, 1])
input_img = np.expand_dims(input_img, 0)
print(input_img.shape)
torch_output = torch_model(torch.from_numpy(input_img)).detach().numpy()
# NCHW to HWC
torch_output = np.squeeze(torch_output, 0)
torch_output = np.clip(torch_output, 0, 255)
torch_output = np.transpose(torch_output, [1, 2, 0]).astype(np.uint8)
cv2.imwrite("assets/out.jpg", torch_output)
test_mode()
# ------------------------------------------------------------
6
generator.conv1.weight torch.Size([64, 3, 9, 9])
generator.conv1.bias torch.Size([64])
generator.conv2.weight torch.Size([32, 64, 1, 1])
generator.conv2.bias torch.Size([32])
generator.conv3.weight torch.Size([3, 32, 5, 5])
generator.conv3.bias torch.Size([3])
init_torch_model success
(1, 3, 256, 256)
动态输入
模型的动态化。出于性能的考虑,各推理框架都默认模型的输入形状、输出形状、结构是静态的。
而为了让模型的泛用性更强,部署时需要在尽可能不影响原有逻辑的前提下,让模型的输入输出或是结构动态化。
上面模型固定了 输入图像维度为 256x256
输入张量维度为 (1, 3, 256, 256)
如何使得模型适配任何图像维度的输入?
导出动态输入
问题-无法修改维度
通过 torch.onnx.export() 的 dynamic_axes 参数来指定动态输入和静态输入
dynamic_axes 的默认值为 None,即默认为静态输入。静态参数无法修改输入数据的维度
如下示例
def mode_export_onnx():
model=init_torch_model()
x = torch.randn(1, 3, 256, 256)
input_names = ["input"] # 定义onnx 输入节点名称
output_names = ["output"] # 定义onnx 输出节点名称
with torch.no_grad():
torch.onnx.export(
model,
x,
"assets/srcnn_cpu.onnx",
input_names=input_names,
output_names=output_names,
opset_version=11,
)
# 导出模型-验证和测试模型
def test_onnx_inter_onnx():
onnx_model = onnx.load("assets/srcnn_cpu.onnx")
try:
onnx.checker.check_model(onnx_model)
except Exception:
print("onnx incorrect")
else:
print("onnx check_model success")
input_img = cv2.imread('assets/images/dog.jpg').astype(np.float32)
input_img = cv2.resize(input_img,(256,320))
# 设置导出维度大小为256,320
# HWC to NCHW
input_img = np.transpose(input_img, [2, 0, 1])
input_img = np.expand_dims(input_img, 0)
print(input_img.shape)
# # 输入维度为(1, 3, 320, 256)
ort_session = onnxruntime.InferenceSession("assets/srcnn_cpu.onnx",
providers=['CPUExecutionProvider']
)
ort_inputs = {'input': input_img}
ort_output = ort_session.run(['output'], ort_inputs)[0]
ort_output = np.squeeze(ort_output, 0)
ort_output = np.clip(ort_output, 0, 255)
ort_output = np.transpose(ort_output, [1, 2, 0]).astype(np.uint8)
print(ort_output.shape)
# 报错 报错信息如下
Traceback (most recent call last):
File "e:\ept_exp_onnx.py", line 136, in <module>
test_onnx_inter_onnx()
File "e:\ept_exp_onnx.py", line 127, in test_onnx_inter_onnx
ort_output = ort_session.run(['output'], ort_inputs)[0]
File "D:\X_Software\Code\miniconda3\envs\py38\lib\site-packages\onnxruntime\capi\onnxruntime_inference_collection.py", line 220, in run
return self._sess.run(output_names, input_feed, run_options)
onnxruntime.capi.onnxruntime_pybind11_state.InvalidArgument: [ONNXRuntimeError] : 2 : INVALID_ARGUMENT : Got invalid dimensions for input: input for the following indices
index: 2 Got: 320 Expected: 256
Please fix either the inputs or the model.
重新定义onnx输出
with torch.no_grad():
torch.onnx.export(
model,
x,
"assets/dynamic_srcnn_cpu.onnx",
input_names=input_names,
output_names=output_names,
opset_version=11,
dynamic_axes = {'input': {0: 'batch_size', 1: 'channel', 2: "height", 3: 'width'},
'output': {0: 'batch_size', 1: 'channel', 2: 'height', 3: 'width'}}
)
# 设置 dynamic_axes
# dynamic_axes 中的 0、2、3 表示相应的维度设置为动态值
def mode_export_dynamic_onnx():
model=init_torch_model()
batch_size = 1
height = 256
width = 256
x = torch.randn(batch_size, 3,height, width)
input_names = ["input"] # 定义onnx 输入节点名称
output_names = ["output"] # 定义onnx 输出节点名称
with torch.no_grad():
torch.onnx.export(
model,
x,
"assets/dynamic_srcnn_cpu.onnx",
input_names=input_names,
output_names=output_names,
opset_version=11,
dynamic_axes = {'input': {0: 'batch_size', 1: 'channel', 2: "height", 3: 'width'},
'output': {0: 'batch_size', 1: 'channel', 2: 'height', 3: 'width'}}
)
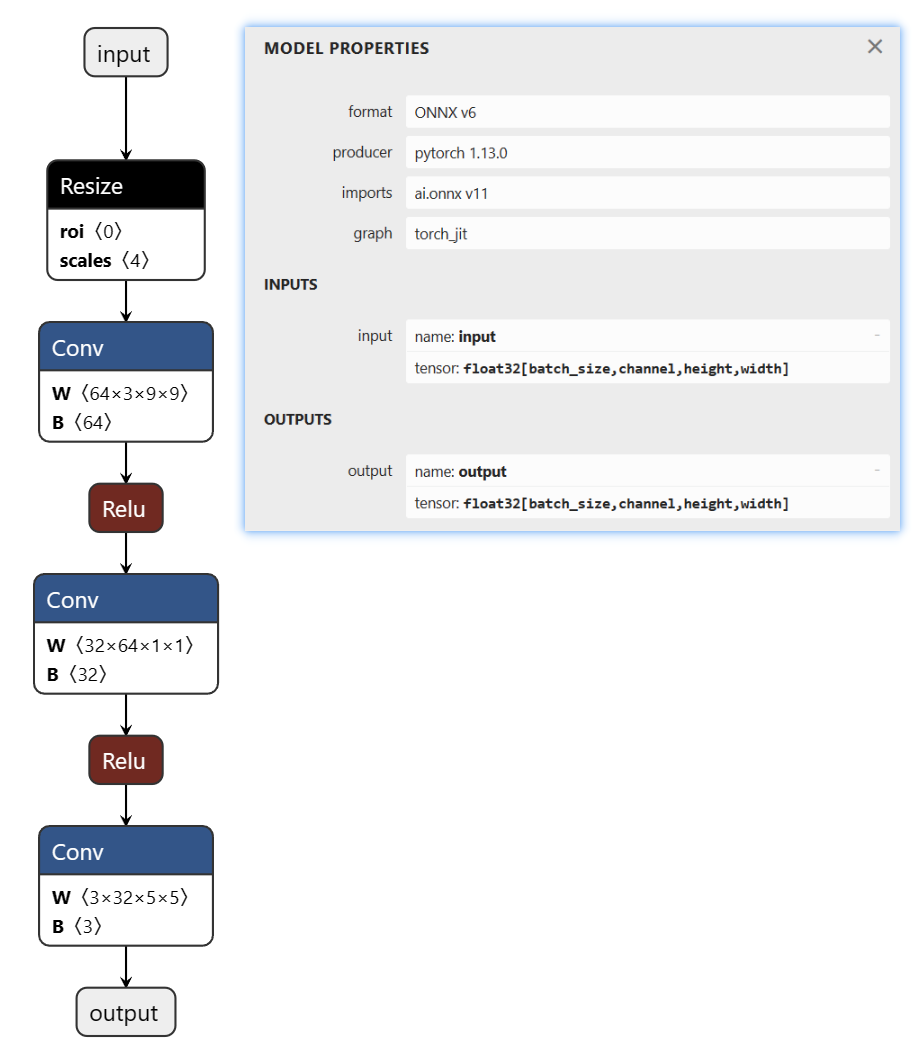
将导出的模型放入到https://netron.app/ 进行可视化
从onnx模型可视化参数来看,input 和 output 都改成了动态维度,支持实时修改输入参数维度
验证导出和测试
def test_dynamic_inter_onnx()
onnx_model = onnx.load("assets\dynamic_srcnn_cpu.onnx")
try:
onnx.checker.check_model(onnx_model)
graph = onnx_model.graph
# print(onnx.helper.printable_graph(onnx_model.graph))
# print(graph.input)
# print(graph.output)
except Exception:
print("onnx incorrect")
else:
print("onnx check_model success")
input_img = cv2.imread('assets/images/dog.jpg').astype(np.float32)
input_img = cv2.resize(input_img,(512,460))
print("input_img transpose pre:", input_img.shape)
# HWC to NCHW
input_img = np.transpose(input_img, [2, 0, 1])
print("input_img transpose pos:", input_img.shape)
input_img = np.expand_dims(input_img, 0)
print("input_img shape:", input_img.shape)
ort_session = onnxruntime.InferenceSession("assets\dynamic_srcnn_cpu.onnx",
providers=['CPUExecutionProvider']
)
ort_inputs = {'input': input_img}
ort_output = ort_session.run(['output'], ort_inputs)[0]
ort_output = np.squeeze(ort_output, 0)
ort_output = np.clip(ort_output, 0, 255)
ort_output = np.transpose(ort_output, [1, 2, 0]).astype(np.uint8)
print("ort_output shape:", ort_output.shape)
cv2.imwrite("assets/out.jpg", ort_output)
# 打印结果
# input_img transpose pre: (460, 512, 3)
# input_img transpose pos: (3, 460, 512)
# input_img shape: (1, 3, 460, 512)
# ort_output shape: (1380, 1536, 3)
多头输入
先来一个简单的案例,新增一个常数输入
import torch.nn as nn
import torch
import numpy as np
import onnx
import onnxruntime
# 定义一个简单的多输入网络
# -----------------------------------#
class MyNet(nn.Module):
def __init__(self, num_classes=10):
super(MyNet, self).__init__()
self.features = nn.Sequential(
# input[3, 28, 28]
nn.Conv2d(3, 32, kernel_size=3, stride=1, padding=1),
# output[32, 28, 28]
nn.BatchNorm2d(32),
nn.ReLU(inplace=True),
nn.Conv2d(32, 64, kernel_size=3, stride=2, padding=1),
# output[64, 14, 14]
nn.BatchNorm2d(64),
nn.ReLU(inplace=True),
nn.MaxPool2d(kernel_size=2)
# output[64, 7, 7]
)
self.fc = nn.Linear(64 * 7 * 7, num_classes)
def forward(self, x, ratio):
# 输入是两个 x,ratio
x = self.features(x)
x = torch.flatten(x, start_dim=1)
x = ratio * self.fc(x)
return x
# 导出ONNX模型函数
# -----------------------------------#
def torch2onnx():
model = MyNet()
model.eval() #使用测试模式
x = torch.randn(1, 3, 28, 28)
ratio = torch.randn(1, 1) # 输入的常数是张量
input_names = ["input1",'input2'] # 配置输入参数,输出参数
output_names = ["output1"]
output_path = 'assets/MyNet.onnx'
torch.onnx.export(
model,
(x,ratio),
output_path,
verbose=False,
opset_version=11,
input_names=input_names,
output_names=output_names,
)
if __name__ == '__main__':
torch2onnx()
输入的第一个参数是张量,传入的第二个参数也必须是张量,要符合pytorch的相关要求。
保证输入的所有参数都是 torch.Tensor 类型
def test_onnx_inter_onnx():
onnx_model = onnx.load("assets/MyNet.onnx")
try:
onnx.checker.check_model(onnx_model)
except Exception:
print("onnx incorrect")
else:
print("onnx check_model success")
x = np.random.randn(1, 3, 28, 28).astype(np.float32)
ratio = np.random.randn(1, 1).astype(np.float32)
print(x.shape)
ort_session = onnxruntime.InferenceSession("assets/MyNet.onnx",
providers=['CPUExecutionProvider']
)
ort_inputs = {"input1": x,"input2": ratio} # 配置输入参数,输出参数
ort_output = ort_session.run(['output1'], ort_inputs)[0]
print(ort_output.shape)
test_onnx_inter_onnx()
多头输出
同样的多头输出,也可以通过定义输出参数名称 实现多头输出
import torch.nn as nn
import torch
import numpy as np
import onnx
import onnxruntime
class Model(torch.nn.Module):
def __init__(self, in_features, out_features, weights1, weights2, bias=False):
super().__init__()
self.linear1 = nn.Linear(in_features, out_features, bias)
self.linear2 = nn.Linear(in_features, out_features, bias)
with torch.no_grad():
self.linear1.weight.copy_(weights1)
self.linear2.weight.copy_(weights2)
def forward(self, x):
x1 = self.linear1(x)
x2 = self.linear2(x)
return x1, x2
def export_onnx():
input = torch.zeros(1, 1, 1, 4)
weights1 = torch.tensor([
[1, 2, 3, 4],
[2, 3, 4, 5],
[3, 4, 5, 6]
],dtype=torch.float32)
weights2 = torch.tensor([
[2, 3, 4, 5],
[3, 4, 5, 6],
[4, 5, 6, 7]
],dtype=torch.float32)
model = Model(4, 3, weights1, weights2)
model.eval() #添加eval防止权重继续更新
# pytorch导出onnx的方式,参数有很多,也可以支持动态size
torch.onnx.export(
model = model,
args = (input,),
f = "assets/two_out.onnx",
input_names = ["input0"],
output_names = ["output0", "output1"],
opset_version = 12)
print("Finished onnx export")
# export_onnx()
def test_onnx_inter_onnx():
onnx_model = onnx.load("assets/two_out.onnx")
try:
onnx.checker.check_model(onnx_model)
except Exception:
print("onnx incorrect")
else:
print("onnx check_model success")
input0 = np.random.randn(1, 1, 1, 4).astype(np.float32)
ort_session = onnxruntime.InferenceSession("assets/two_out.onnx",
providers=['CPUExecutionProvider']
)
ort_inputs = {"input0": input0} # 配置输入参数,输出参数
ort_output = ort_session.run(['output0','output1'], ort_inputs)
print(ort_output)
test_onnx_inter_onnx()
# -----------------------------------------------------------------------------------
onnx check_model success
[array([[[[ 8.880694, 12.145373, 15.41005 ]]]], dtype=float32),
array([[[[12.145373, 15.41005 , 18.674728]]]], dtype=float32)]
参考资料
知乎-OpenMMLab-模型部署入门教程(一):模型部署简介
知乎OpenMMLab-模型部署入门教程(二):解决模型部署中的难题
知乎-OpenMMLab-模型部署入门教程(三):PyTorch 转 ONNX 详解
正确导出onnx|onnx结构|编辑onnx各类节点|onnx算子编写|复杂后处理的添加|onnx形状推理
标签:onnx,ort,torch,导出,input,维度,output,model From: https://www.cnblogs.com/tian777/p/17995361