# DeFeeNet: Consecutive 3D Human Motion Prediction with Deviation Feedback #paper
1. paper-info
1.1 Metadata
- Author:: [[Xiaoning Sun]], [[Huaijiang Sun]], [[Bin Li]], [[Dong Wei]], [[Weiqing Li]], [[Jianfeng Lu]]
- 作者机构:: njust.edu.cn
- Keywords:: #HMP
- Journal:: #CVPR
- Date:: [[2023-04-14]]
- 状态:: #Doing
Sun X, Sun H, Li B, et al. DeFeeNet: Consecutive 3D Human Motion Prediction with Deviation Feedback[J]. arXiv preprint arXiv:2304.04496, 2023.
1.2. Abstract
Let us rethink the real-world scenarios that require human motion prediction techniques, such as human-robot collaboration. Current works simplify the task of predicting human motions into a one-off process of forecasting a short future sequence (usually no longer than 1 second) based on a historical observed one. However, such simplification may fail to meet practical needs due to the neglect of the fact that motion prediction in real applications is not an isolated observe then predict unit, but a consecutive process composed of many rounds of such unit, semi-overlapped along the entire sequence. As time goes on, the predicted part of previous round has its corresponding ground truth observable in the new round
, but their deviation in-between
is neither exploited nor able to be captured by existing isolated learning fashion. In this paper, we propose DeFeeNet, a simple yet effective network that can be added on existing one-off prediction models to realize deviation perception and feedback
when applied to consecutive motion prediction task. At each prediction round, the deviation generated by previous unit is first encoded by our DeFeeNet, and then incorporated into the existing predictor to enable a deviation-aware prediction manner, which, for the first time, allows for information transmit across adjacent prediction units. We design two versions of DeFeeNet as MLP-based and GRU-based, respectively. On Human3.6M and more complicated BABEL, experimental results indicate that our proposed network improves consecutive human motion prediction performance regardless of the basic model.
2. Story
随着时间的推移,人体姿势可能变得难以预测,目前的工作将实际需求抽象为一个简化的任务,即学习“观察几帧然后预测后续帧”,预测长度大多设置为≤1秒。将该简易的过程看做一个“observe and predict”单元,然而,这个单元实际上并不适用于需要在人-机器人/机器长期共存期间对人类进行连续观察和预测的现实。虽然从直观上看,沿着时间逐轮滑动这样的单元可以大致满足连续预测的需要,但一个被忽略的事实是每一轮预测单元都是以半重叠结构排列的(见Fig1)。
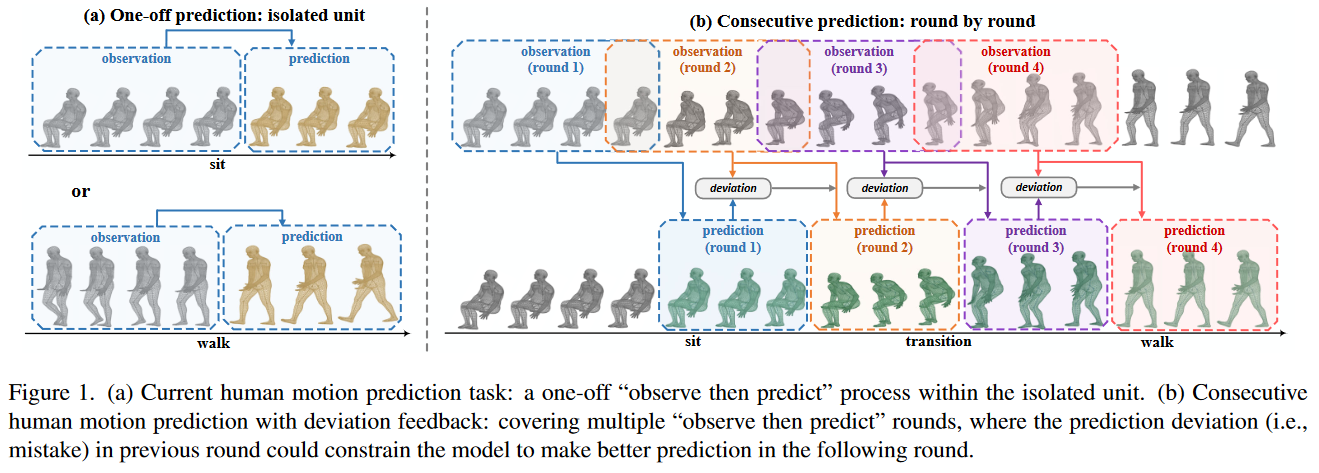
Fig.1
为了能够检测到预测时候的偏差,作者利用半重叠的多轮单元结构,提供了一种在相邻预测单元之间传递偏差反馈的方式。
3. Methods
3.1 问题定义
a motion sequence \(S=[s_1,s_2,..,s_L]\) ,在\(S\)中,定义多个round,每一个round的长度为\(N+T\),最开始的round\(R_1=s_{1:N+T}\);第\(r\)个 round \(R_r=s_{1+(r-1)T:N+T+(r-1)T}\)
其中\(N>=T\), \(R=[R_1,R_2,...,R_r,...]\),\(R_r=[X_{r, Y_r}]=[x_{r,1},...,x_{r,N},y_{r,1},...,y_{r,T}]\) ,可以推导出\(x_{r,N-T:N}=y_{r-1,1:T}\)
每一轮的偏差预测可以用公式表示为:
\[D_{r-1}=f_d(x_{r,N-T:N},\hat{y}_{r-1,1:T}) \]3.2 Deviation-Aware Prediction
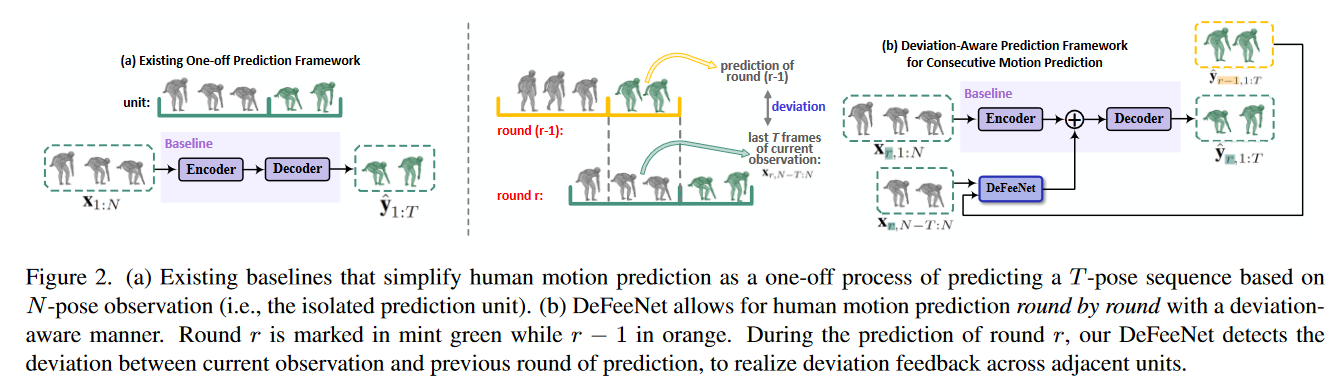
Fig.1 模型流程说明
3.3 Architecture
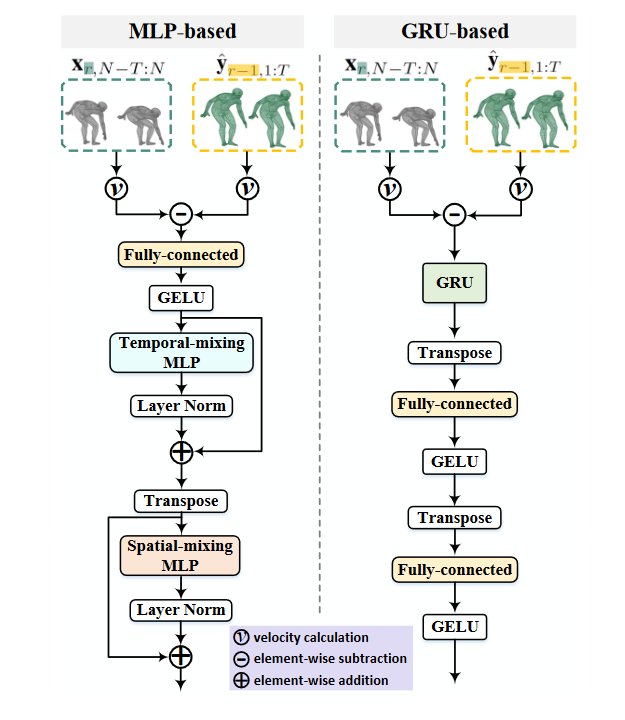
Fig.2 DeFeeNet模型结构
提出了两种模型,一种基于MLP,一种基于GRU。
4. Experiments
- datasets
- HUman3.6M
- BABEL :新提出的数据库,包含语义标签。
- Evaluation Metric
- MPJPE
5. 总结
本文的思想很简单,通过不断反馈的方式,不断修正错误。
标签:deviation,mathbf,sun,prediction,motion,CVPR,DeFeeNet,round From: https://www.cnblogs.com/guixu/p/17351521.html