# Trace and Pace: Controllable Pedestrian Animation via Guided Trajectory Diffusion #paper
1. paper-info
1.1 Metadata
- Author:: [[Davis Rempe]], [[Zhengyi Luo]], [[Xue Bin Peng]], [[Ye Yuan]], [[Kris Kitani]], [[Karsten Kreis]], [[Sanja Fidler]], [[Or Litany]]
- 作者机构::
- Keywords:: #TrajectoryPrediction , #RL , #Diffusion
- Journal:: #CVPR
- Date:: [[2023-04-04]]
- 状态:: #Doing
- 网址:: https://research.nvidia.com/labs/toronto-ai/trace-pace/supp.html
Rempe D, Luo Z, Peng X B, et al. Trace and Pace: Controllable Pedestrian Animation via Guided Trajectory Diffusion[J]. arXiv preprint arXiv:2304.01893, 2023.
1.2. Abstract
We introduce a method for generating realistic pedestrian trajectories
and full-body animations
that can be controlled to meet user-defined goals. We draw on recent advances in guided diffusion modeling
to achieve test-time controllability
of trajectories, which is normally only associated with rule-based systems. Our guided diffusion model allows users to constrain trajectories through target waypoints, speed, and specified social groups while accounting for the surrounding environment context. This trajectory diffusion model is integrated with a novel physics-based humanoid controller
to form a closed-loop, full-body pedestrian animation system capable of placing large crowds in a simulated environment with varying terrains. We further propose utilizing the value function
learned during RL training of the animation controller to guide diffusion to produce trajectories better suited for particular scenarios such as collision avoidance and traversing uneven terrain. Video results are available on the project page at https://nv-tlabs.github.io/trace-pace .
本文针对行人轨迹预测的生成和人体动画问题,根据最近大火的guided diffusion modeling
, 结合RL,提出了一种可以控制轨迹生成且可以模拟的方法。
2. Story
目标:控制高level的人类行为(2D 的轨迹坐标),这里的控制包括通过目标点控制,或者其他启发式控制(行人,人群,交通工具),但是之前的方法都无法生成自然的人体轨迹,因为之前的方法大多数为learning-based
方法,会出现一些不合理的动作(例如:与障碍物碰撞,或者与行人碰撞)。作者想实现更真实的轨迹生成(agent-agent and agent-environment interactions),并且能够结合轨迹给出模拟动画。
虽然之前的方法已经提出task-spectific planners
用于控制physics-based methods
来生成人体动作,但是基于任务的方法针对不同的任务需要单独进行训练,不具有泛化性。
作者开发了一种数据驱动、可控并与基于物理的动画系统紧密集成的轨迹生成模型,用于全身行人模拟。主要包括两部分:
- Trajectory Diffusion Model for Controllable PEdestrians(TRACE):能够根据条件生成合理且真实的轨迹。
- Pedestrain Animation Controller(PACER): 能够模拟人体全身结构,根据轨迹产生动作。
3. Method
3.1 Controllable Trajectory Diffusion
Problem Setting: 根据条件,生成可控制的人体轨迹。控制分为两类:
- 基于用户的:goal waypoint(给定目标点,生成经过目标点的轨迹),social distance(社交距离),social group(社会群体)。
- 基于物理条件的:防止与障碍物进行碰撞,防止agent-agent之间的碰撞。
Formulate
agent对应的轨迹为\(\tau_s=[s_{t+1},s_{t+2}, .., s_{t+T_f}]\) , \(s\)为特定时间\(t\)的状态\(s=[x,y,\theta, \mathcal{v}]^T\) ;\(\tau_s\)都是由一系列的action\(\tau_a=[a_{t+1},a_{t+2},...,a_{t+T_f}]\)产生的,action \(a=[\overset{.}{\mathcal{v}}, \overset{.}{\theta} ]^T\)
- \((x,y)\)为2D位置坐标点
- \(\theta\):头的偏角
- \(\mathcal{v}\):速度
- \(\overset{.}{a}\):加速度
- \(\overset{.}{\theta}\):偏航率
轨迹可以通过给定初始状态和action得到:
\[\tau_s=f(s_t,\tau_a);\;f:dynamics\; model \]conditioning context \(C=\{x^{ego}, X^{neigh}, \mathcal{M}\}\)
- \(\mathbf{x}^{\mathrm{ego}}=\left[\begin{array}{lll}\mathbf{s}_{t-T_{p}} & \ldots & \mathbf{s}_{t}\end{array}\right]\) :ego pedestrain 自我行人,当前agent对应的历史轨迹
- \(X^{\text {neigh }}=\left\{\mathrm{x}^{\mathrm{i}}\right\}_{i=1}^{N}\):\(N\)个neighboring pedestrians
- \(\mathcal{M}\in \mathbb{R}^{H\times W \times C}\): 对当前agent周围环境的栅格化的裁剪
3.1.1 Trajectory Diffusion Model
本文通过事后条件classifier-guided
+ 事前修改classifier-free
的扩散模型的方式,来起到条件生成的效果。
前向扩散过程:
逆向扩散过程:
\[p_{\phi}\left(\boldsymbol{\tau}^{k-1} \mid \boldsymbol{\tau}^{k}, C\right):=\mathcal{N}\left(\boldsymbol{\tau}^{k-1} ; \boldsymbol{\mu}_{\phi}\left(\boldsymbol{\tau}^{k}, k, C\right), \boldsymbol{\Sigma}_{k}\right) \]Training and Classifier-Free Sampling
首先采用事先训练的方式训练扩散模型,但是不同于一般的条件扩散模型,通过对条件进行随机剪裁,可以得到有条件和无条件的训练结果。
用权重系数\(w\)控制条件对预测结果的影响程度。当\(w=0\)时,纯条件扩散模型;当\(w=-1\)时,为无条件扩散模型。--->通过这种训练方式,不仅能够更好的控制,而且泛化能力会提高,并且可以兼容不同的数据集(一些数据集没有环境信息)
Architecture
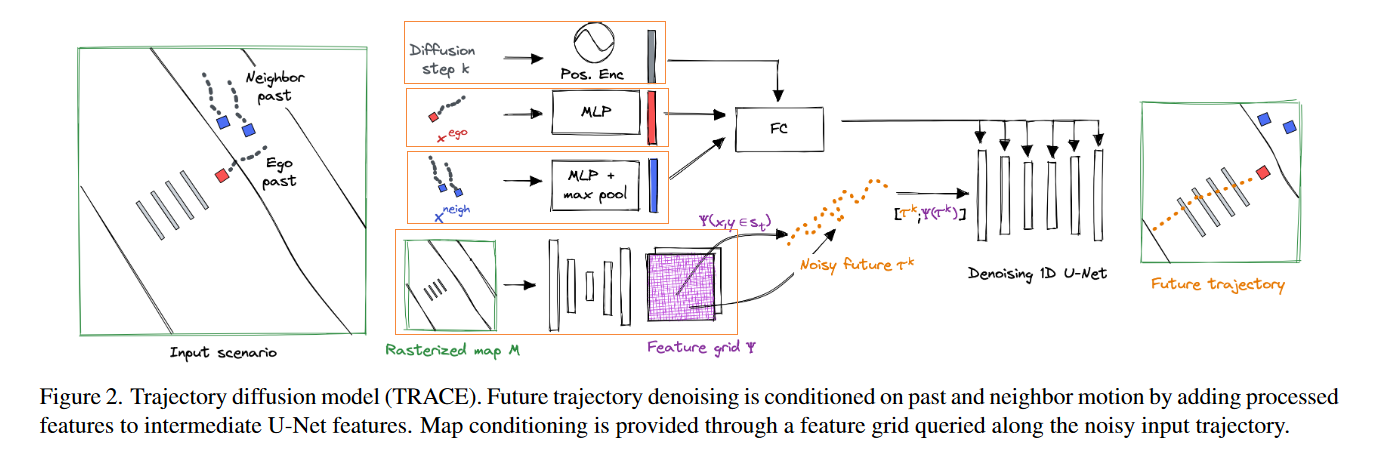
Fig.1TRACE中的模型框架
整体结构采用Unet的方式,为了将条件引入模型,作者对不同的条件使用了不同的加入方式:
- step k(k步扩散的结果),ego past \(x^{ego}\),neighbor past\(X^{neigh}\),通过FC embedding后加入到每一个上采样,下采样卷积块中。
- 对于map \(\mathcal{M}\),使用2D卷积编码得到
a feature grid
,网格的每一个点都对应一个高维的特征。每一个2D position \((x,y)\in \tau^k\)插入到对应的网格特征中,联合得到Unet的输入。
3.1.2 Controllability through Clean Guidance
将条件扩散模型训练完毕后,通过事后修改classifier-guidance
的方式,来控制生成。
常规的方式是对生成的\(\mu\)进行打扰来引导生成:
\[\tilde{\boldsymbol{\mu}}=\boldsymbol{\mu}-\alpha \boldsymbol{\Sigma}_{k} \nabla_{\boldsymbol{\mu}} \mathcal{J}(\boldsymbol{\mu}) \]\(\mathcal{J}(\tau)\): a guidance loss funcion 用于衡量该轨迹为目标轨迹的程度。(分类器)
对于上面这种干扰\(\mu\)的方式,优化损失函数时必须在不同的噪声水平下进行训练,损失函数可能会遇到数值问题。
为了避免这种问题,作者提出了reconstruction guidance
,直接对clean model prediction
\(\hat{\tau}^0\) 进行作用,计算方式如下:
注1:为了理解这种方式的可行性,得了解扩散模型逆向过程的原理,预测的推理过程\(使用x_t去预测x_0,-->考虑到分布的形式-->预测\mu-->预测\theta\)
注2:梯度是根据噪声输入轨迹 \(\tau^k\)而不是干净的\(\hat{\tau}^0\)进行评估的,这需要通过去噪模型进行反向传播。
3.2 Physics-Based Pedestrian Animation
为了实现全身行人模拟,作者设计了行人动画控制器 (PACER) 以在物理模拟器中执行 TRACE 生成的二维轨迹。
Background: Goal-Conditioned RL
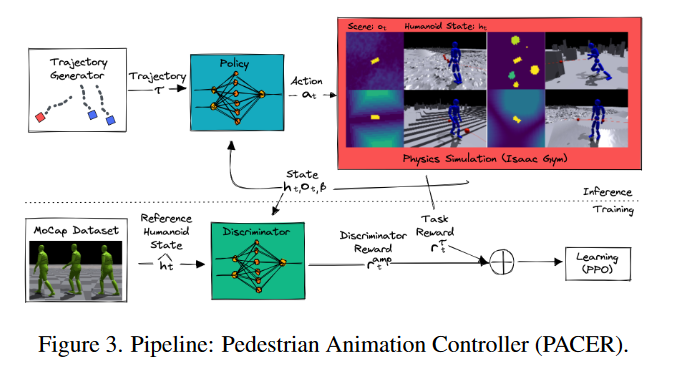
Fig. 2PACER 模型结构
TRACE 建立在 a goal-conditioned reinforcement learning framework上,policy\(\pi_{PACER}\)通过建立在\(\tau_s\)上。结合强化学习,将该任务描述为一个Markov Decision process
\(\mathcal{M}=<S,A,\tau,R, \gamma>\) ,采用PPO
算法进行优化。
Terrain, Social, and Body Awareness
为了创建一个可以在逼真的 3D 场景中模拟人群的控制器,人形机器人必须具有地形感知能力、对其他代理人的社交意识并支持不同的身体类型。创建的人形机器人符合SMPL的力学结构,control policy的计算方式为:
\(h_t\):角色状态
\(o_t\in\mathbb{R}^{64\times64\times3}\):环境特征
\(\beta\):人体结构类型
\(\tau_s\):轨迹
为了允许社会意识,附近的类人动物被表示为一个长方体并呈现在全局高度图上。这样,每个类人动物都将其他人视为需要避免的动态障碍。
Realistic Motion through Adversarial Learning
为了能够产生最优的policy\(\pi_{PACER}\)[1. 符合2D轨迹,2.具有真是的行人动作],作者采用Adversarial Motion Prior(AMP) ,AMP 使用运动鉴别器来鼓励策略生成类似于人类演员记录的运动剪辑数据集中包含的运动模式的运动、该判别器Discriminator\(D(h_{t-10:t},a_t)\)通过一种特殊的动作类型reward\(r_t^{amp}\)来训练policy。
总的reward:
\[r_{t}=r_{t}^{\text {amp }}+r_{t}^{\tau}+r_{t}^{\text {energy }} \]3.3 Contrrollable Pedestrian Animation System
将TRACE和PACER结合得到端到端的行人动画系统。这两者独立训练。在模拟时采用一个反馈循环:PACER 在 TRACE(将来自 PACER 的过去角色运动作为输入) 重新规划之前遵循规划轨迹 2 秒。通过将 PACER 的地形和社会意识与避免碰撞指导相结合,高级和低级系统都具有任务感知能力,并协同工作以防止碰撞和跌倒。
Value Function as Guidance
在RL训练过程中,利用可学习的价值函数value function来引导trajectory diffusion model。价值函数预测预期的未来回报,并了解身体姿势和周围的地形和代理人。
4. Experiments
实验过程和结果请看原文和拓展材料link
5. 总结
这是我目前为止看过的最精彩的paper,理论完备,效果惊艳,将扩散模型的两种条件方式利用的很好,并且结合强化学习,提升了模型灵活度。强化学习并不是直接作用于扩散模型,而是通过产生条件,来控制扩散模型的输出。同时扩散模型的输出又会影响强化学习产生action的过程。两者互相反馈。
问题:扩散模型的采样时间过长。
future:如何将扩散模型利用到low-level full-body character control.
参考文献
【1】Peng X B, Ma Z, Abbeel P, et al. Amp: Adversarial motion priors for stylized physics-based character control[J]. ACM Transactions on Graphics (TOG), 2021, 40(4): 1-20.
标签:Diffusion,Controllable,via,tau,boldsymbol,right,mathcal,轨迹,left From: https://www.cnblogs.com/guixu/p/17353541.html