我们介绍了一种名为可组合扩散(CoDi)的新型生成模型,能够从任意输入模态的任意组合中生成任意组合的输出模态,例如语言、图像、视频或音频。与现有的生成人工智能系统不同,CoDi能够同时生成多个模态,并且其输入不限于文本或图像等子集模态。尽管许多模态组合缺乏训练数据集,我们提出在输入和输出空间中对模态进行对齐。这使得CoDi能够自由地根据任意输入组合进行条件生成,并生成任意模态组合,即使它们在训练数据中不存在。CoDi采用一种新颖的可组合生成策略,通过在扩散过程中建立共享的多模态空间,实现模态的同步生成,例如时间对齐的视频和音频。高度可定制和灵活的CoDi实现了强大的联合模态生成质量,并且在单模态合成方面优于或与最先进的单模态技术持平。
CoDi: Any-to-Any Generation via Composable Diffusion
Zineng Tang1*, Ziyi Yang2†, Chenguang Zhu2, Michael Zeng2, Mohit Bansal1† 1University of North Carolina at Chapel Hill, 2Microsoft Azure Cognitive Services Research * Work done at Microsoft internship and UNC. †Corresponding Authors Paper Code Demo Video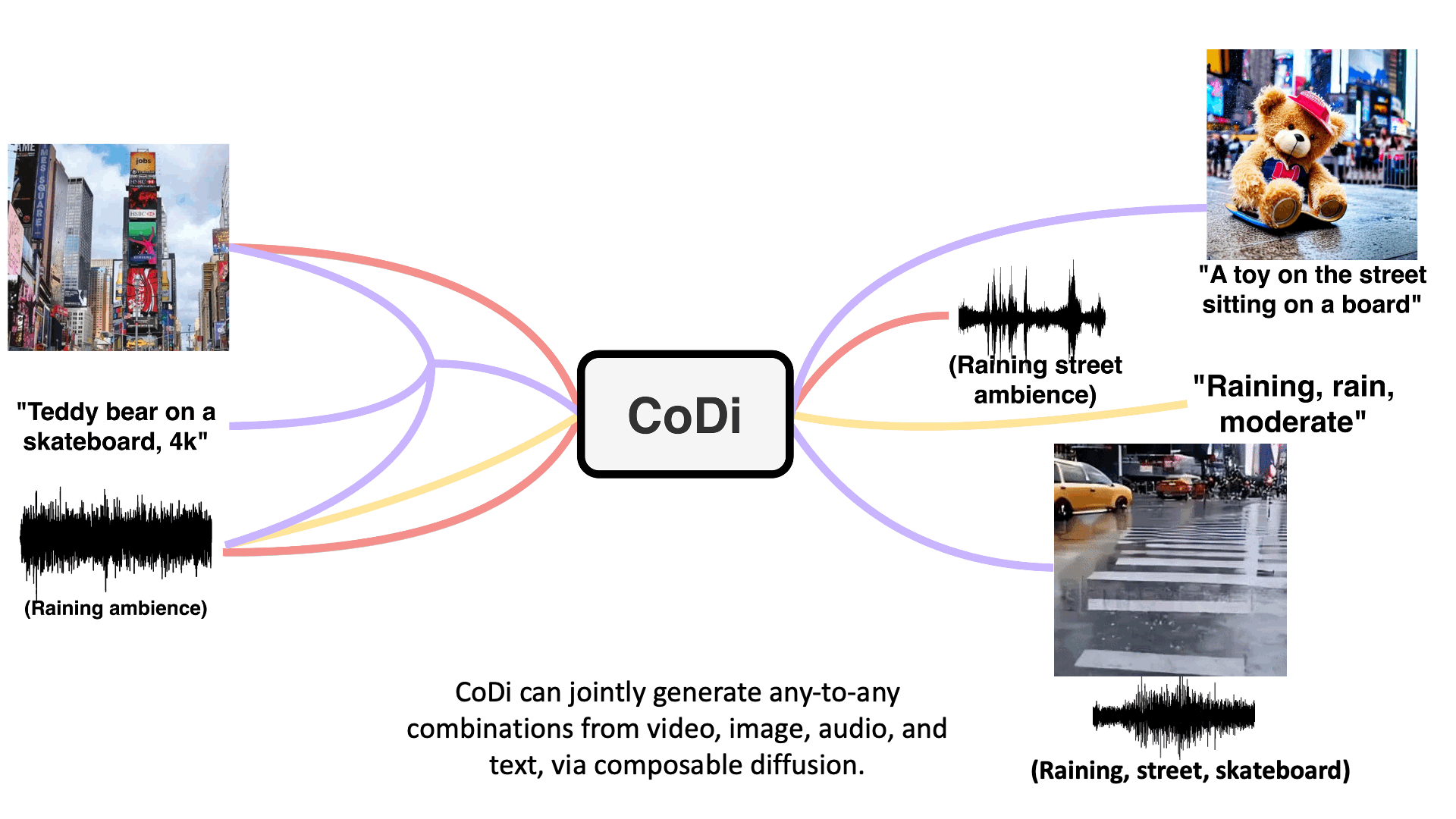
Abstract
We present Composable Diffusion (CoDi), a novel generative model capable of generating any combination of output modalities, such as language, image, video, or audio, from any combination of input modalities. Unlike existing generative AI systems, CoDi can generate multiple modalities in parallel and its input is not limited to a subset of modalities like text or image. Despite the absence of training datasets for many combinations of modalities, we propose to align modalities in both the input and output space. This allows CoDi to freely condition on any input combination and generate any group of modalities, even if they are not present in the training data. CoDi employs a novel composable generation strategy which involves building a shared multimodal space by bridging alignment in the diffusion process, enabling the synchronized generation of intertwined modalities, such as temporally aligned video and audio. Highly customizable and flexible, CoDi achieves strong joint-modality generation quality, and outperforms or is on par with the unimodal state-of-the-art for single-modality synthesis.
Model Architecture
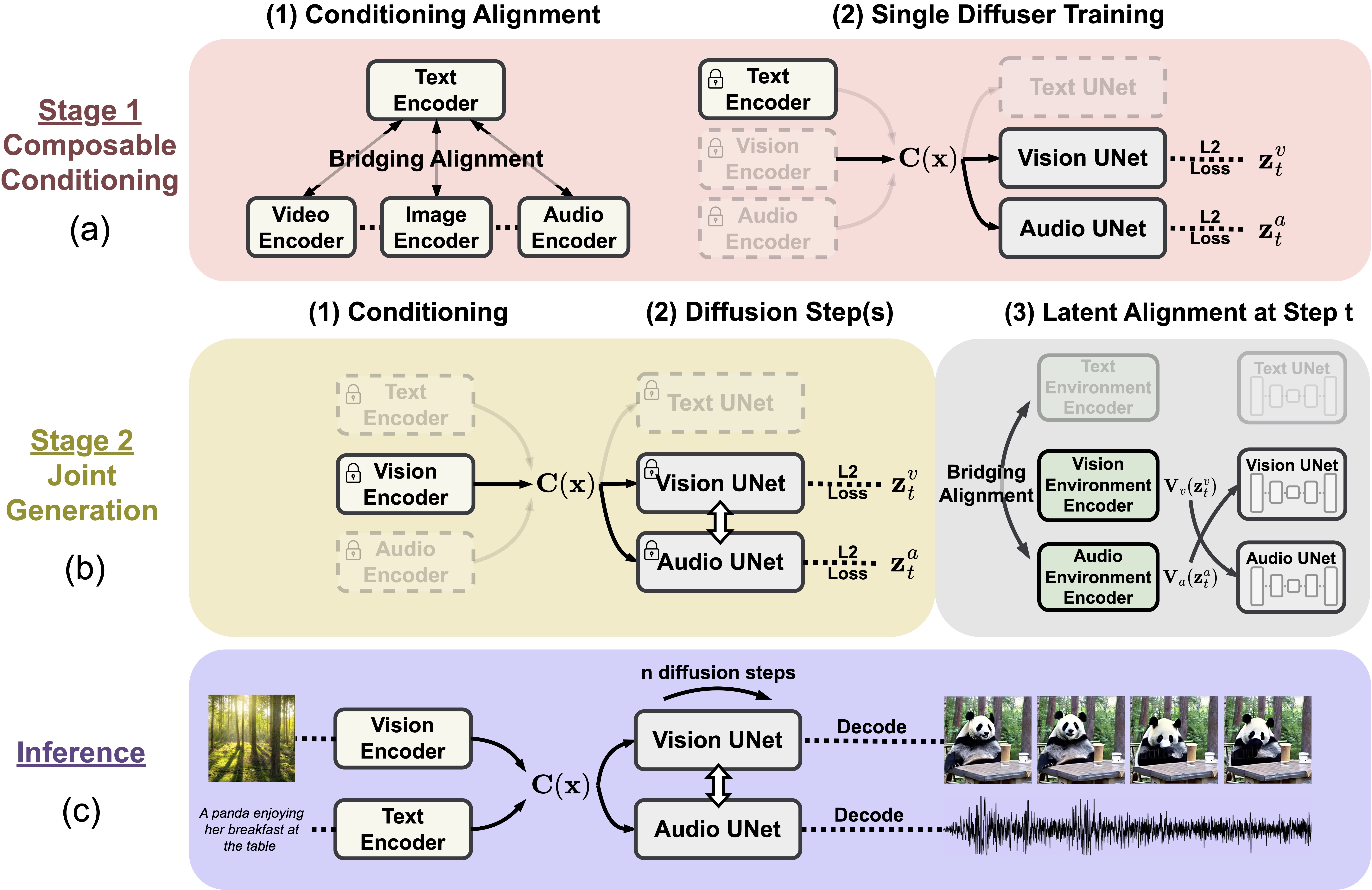
Multi-Outputs Joint Generation
Model takes in single or multiple prompts including video, image, text, or audio to generate multiple aligned outputs like video with accompanying sound.
Text + Image + Audio → Video + Audio
"Teddy bear on a skateboard, 4k, high resolution"
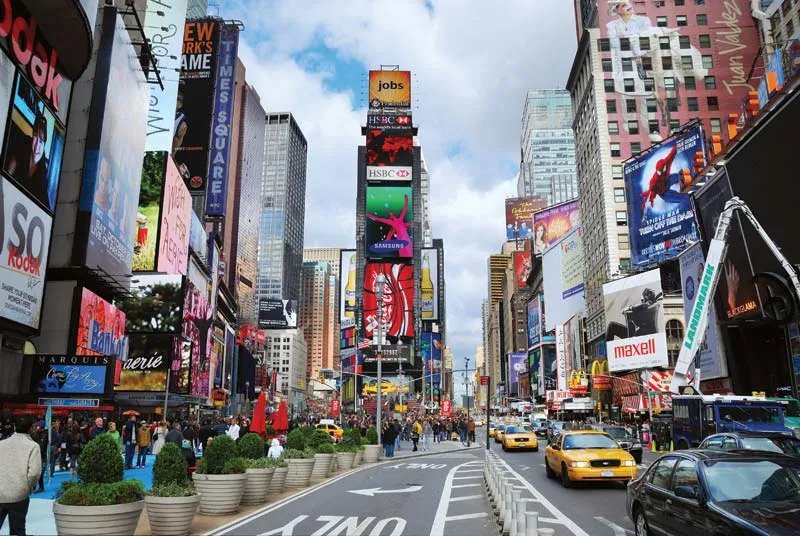
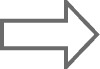
Text + Audio + Image → Text + Image
"Teddy bear on a skateboard, 4k, high resolution"
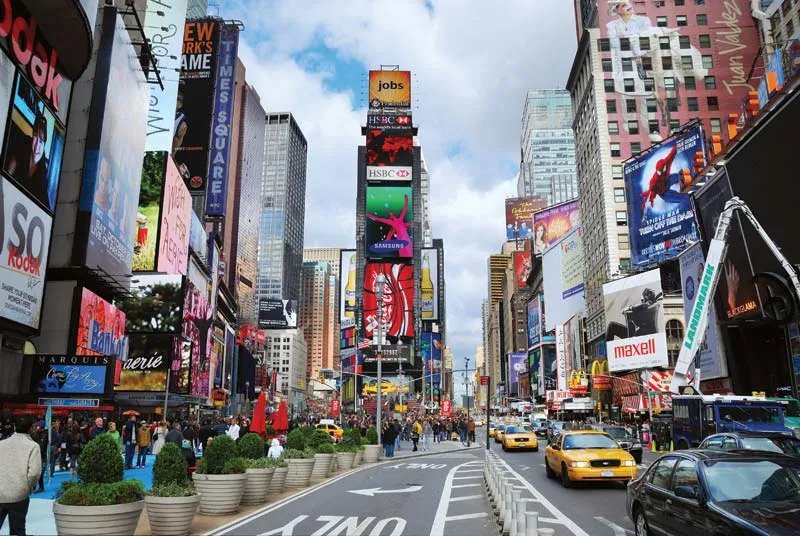
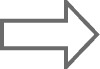
"A toy on the street sitting on a board"
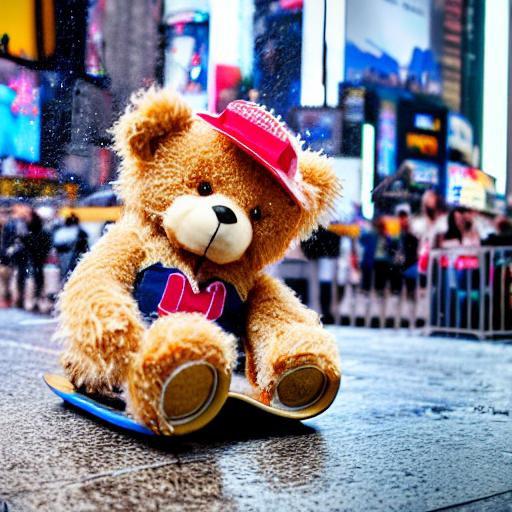
Audio + Image → Text + Image
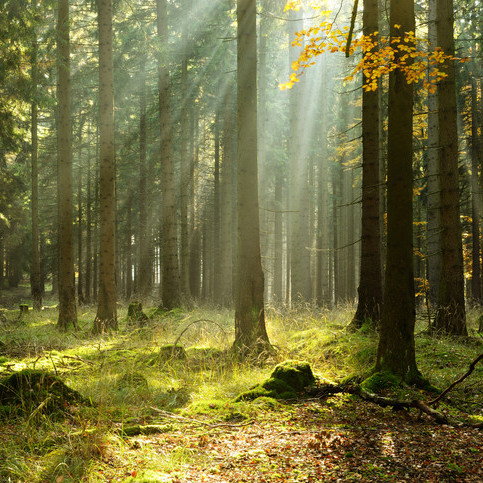
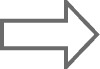
"Playing piano in a forest."
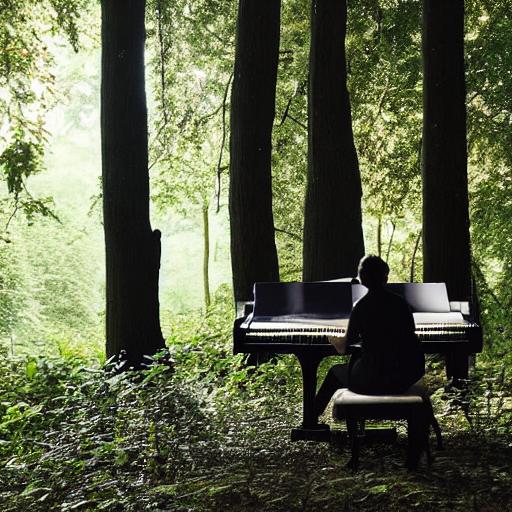
Text + Image → Text + Image
"Cyberpunk vibe."
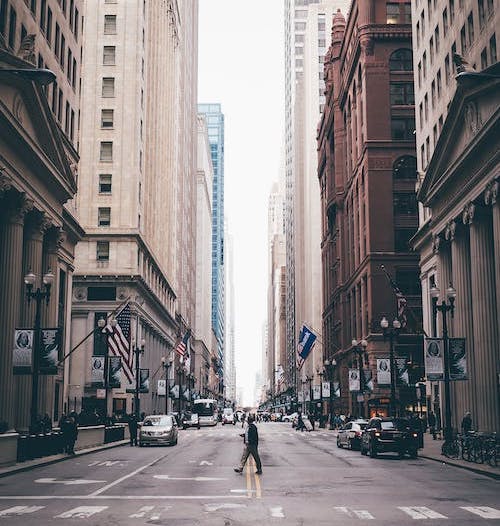
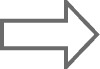
"Cyberpunk, city, movie scene, retro ambience."
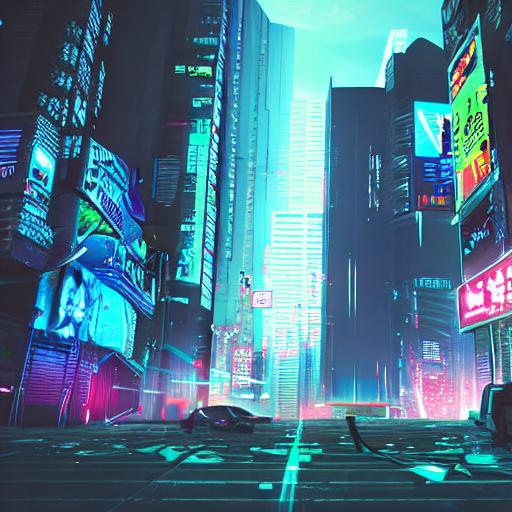
Text → Video + Audio
"Fireworks in the sky."
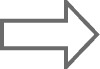
Text → Video + Audio
"Dive in coral reef."
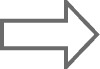
Text → Video + Audio
"Train coming into station."
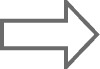
Text → Text + Audio + Image
"Sea shore sound ambience."
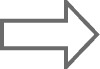
"Wave crashes the shore, sea gulls."
Text → Text + Audio + Image
"Street ambience."
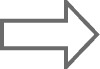
"Noisy street, cars, traffics.."
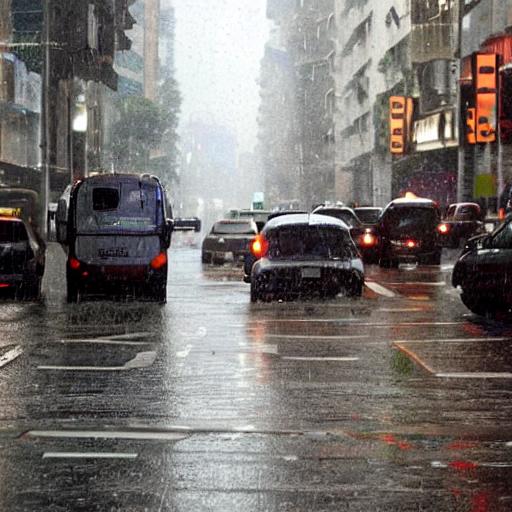
Multiple Conditioning
Model takes in multiple inputs including video, image, text, or audio to generate outputs.
Text + Audio → Image
"Oil painting, cosmic horror painting, elegant intricate artstation concept art by craig mullins detailed"
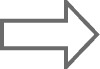
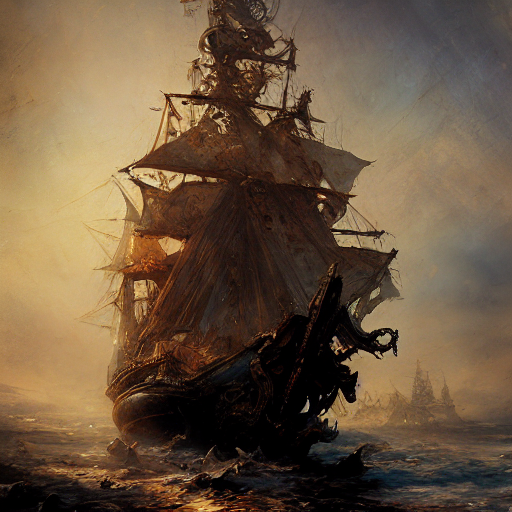
Text + Image → Image
"Gently flowers in a vase, still life, by Albert Williams"
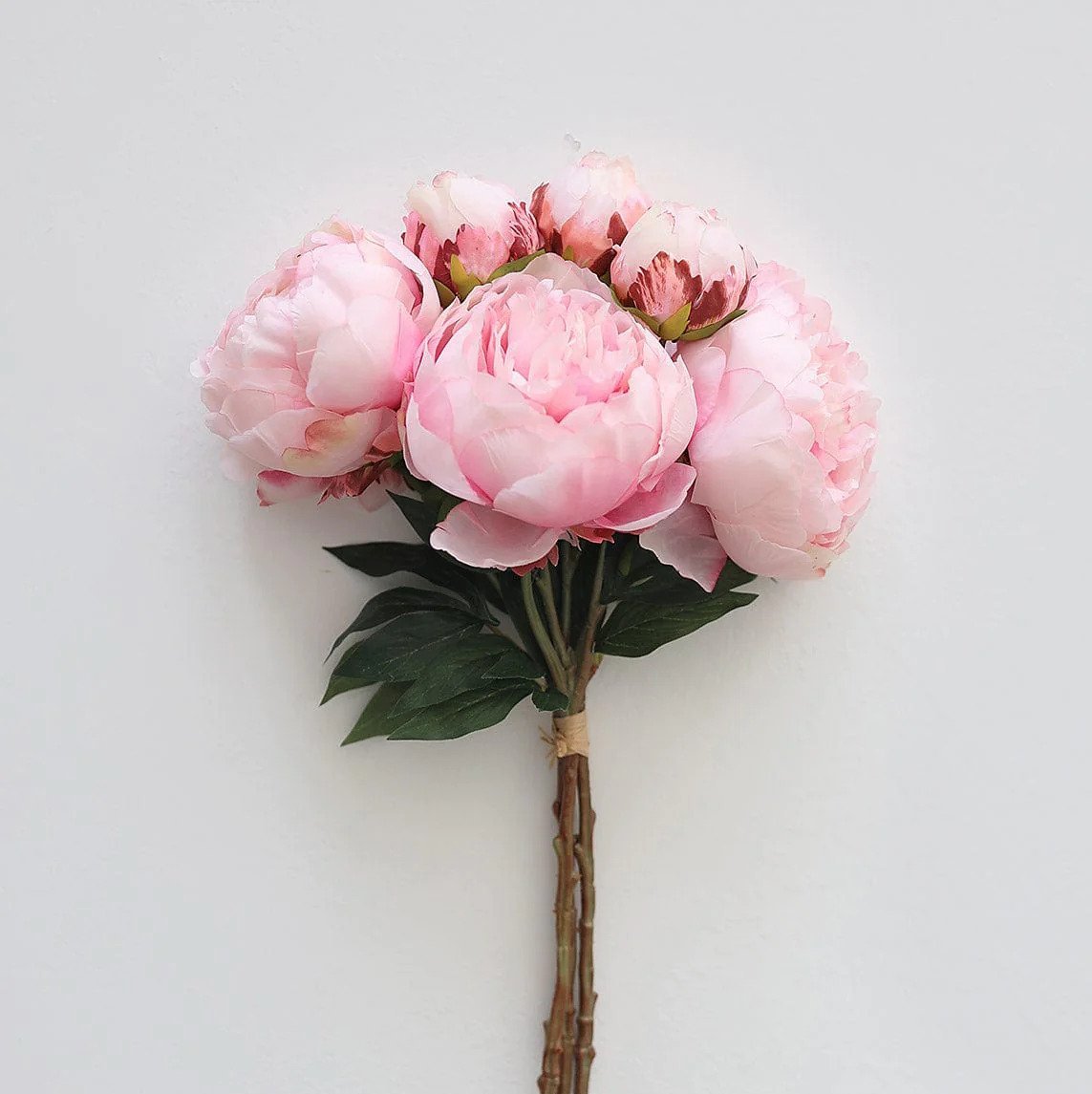
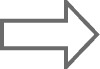
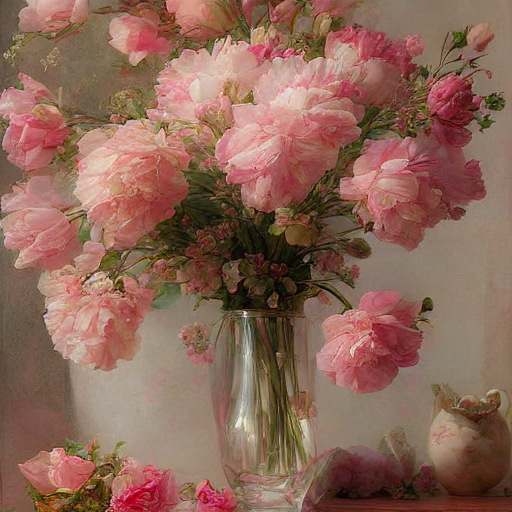
Text + Audio → Video
"Forward moving camera view."
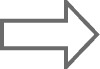
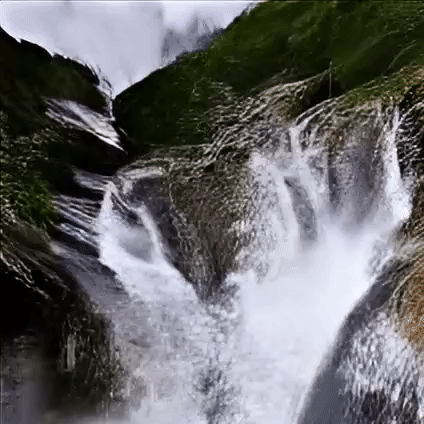
Text + Image → Video
"Red gorgonian and tropical fish."
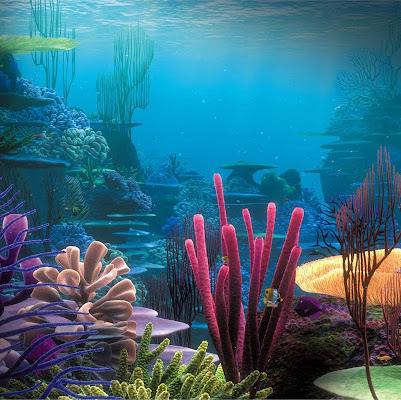
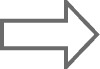
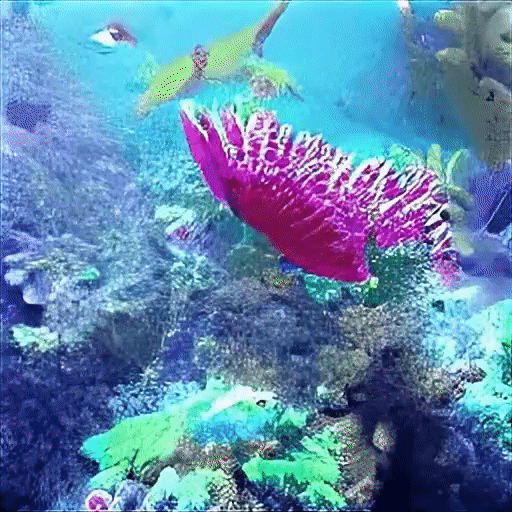
Text + Image → Video
"Eating on a coffee table."
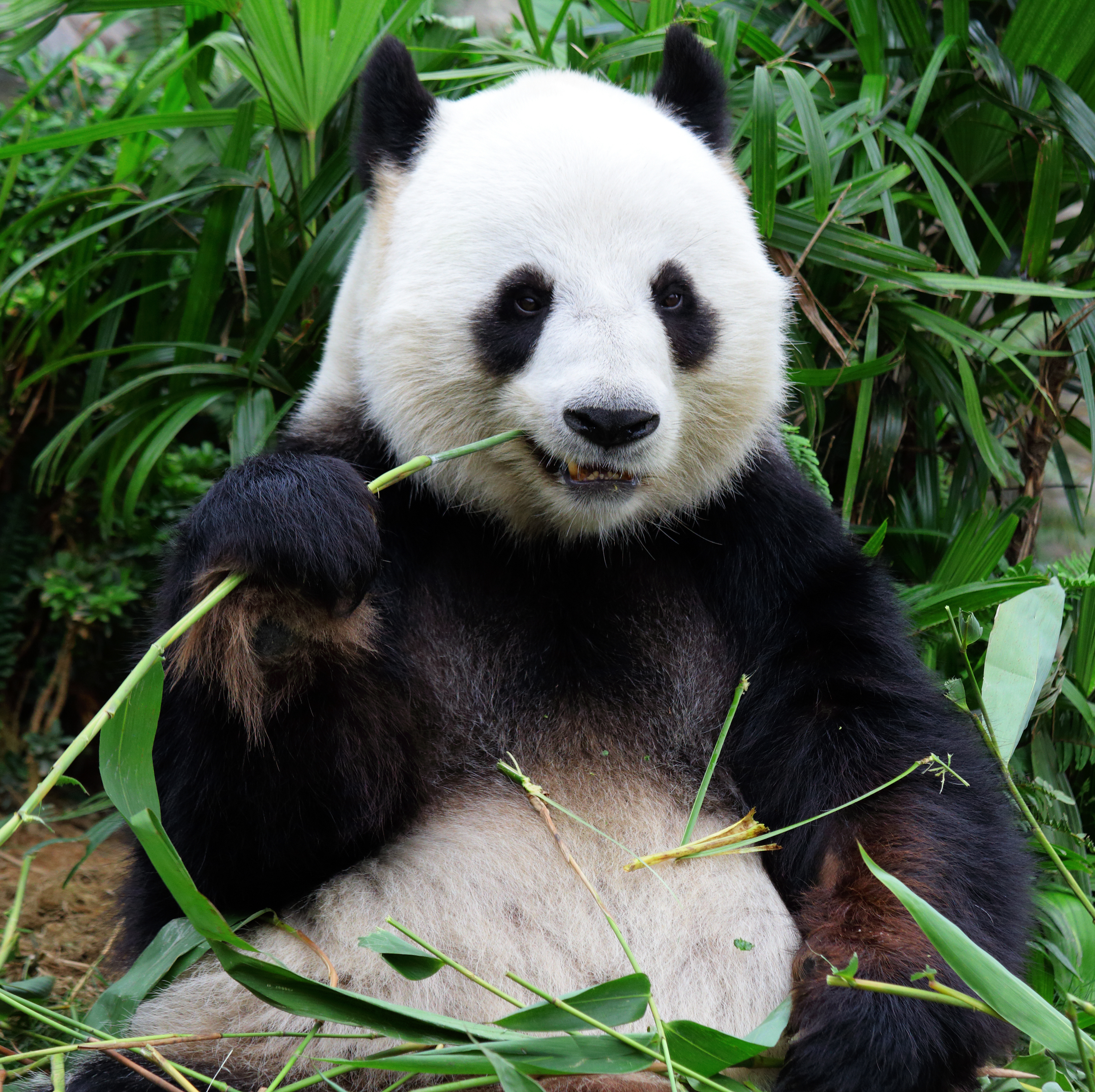
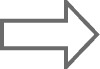
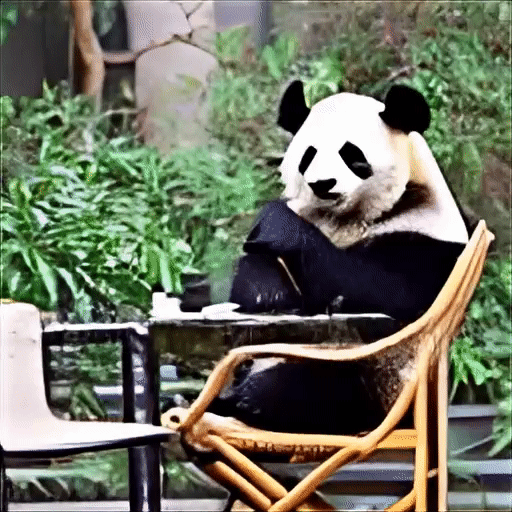
Video + Audio → Text
1.00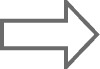
"Panda eating bamboo, people laughing."
Image + Audio → Audio
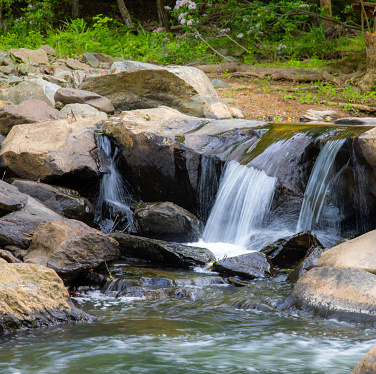
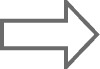
Text + Image → Audio
"Horn, blow whistle"
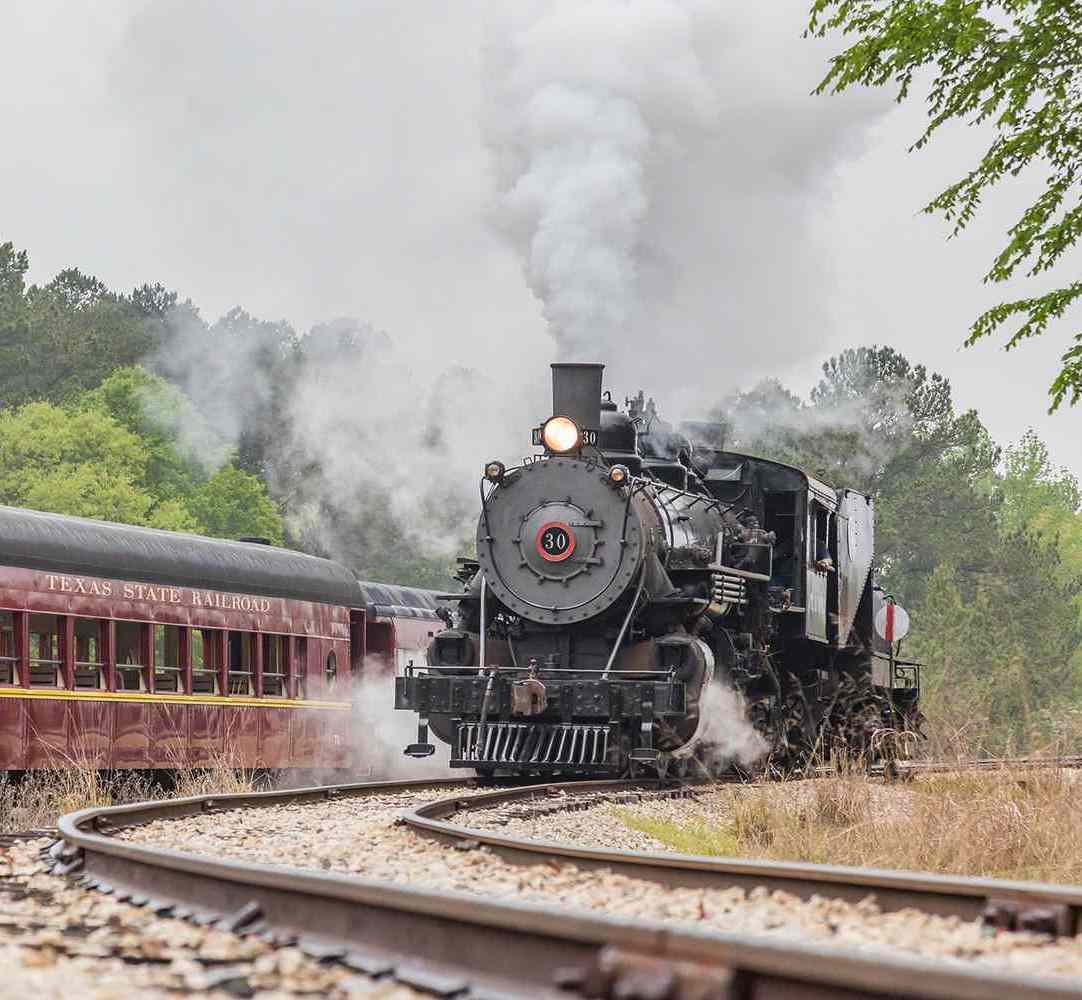
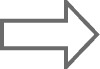
Single-to-Single Generation
Model takes in a single prompt including video, image, text, or audio to generate a single output.
Text → Image
"Concept art by sylvain sarrailh of a haunted japan temple in a forest"
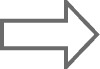
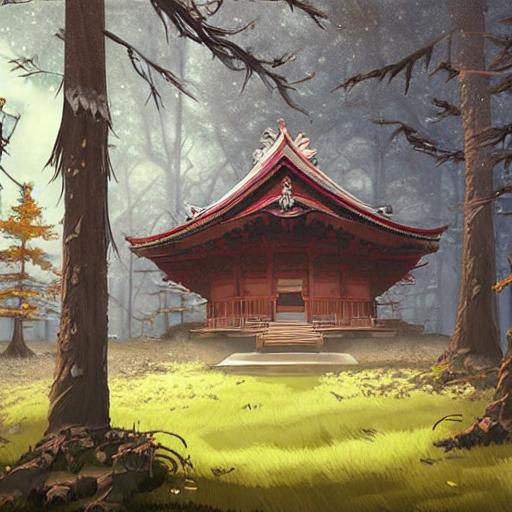
Audio → Image
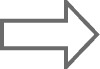
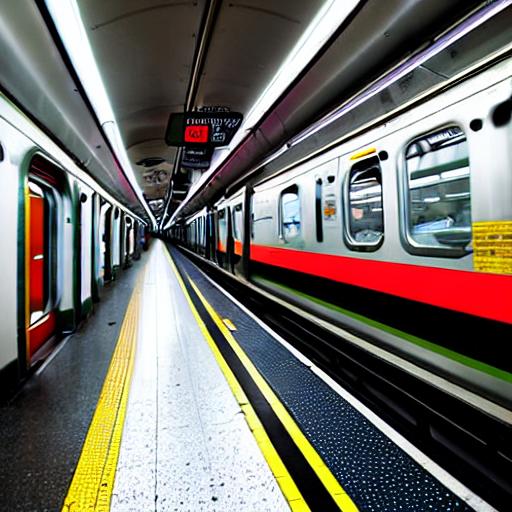
Image → Video
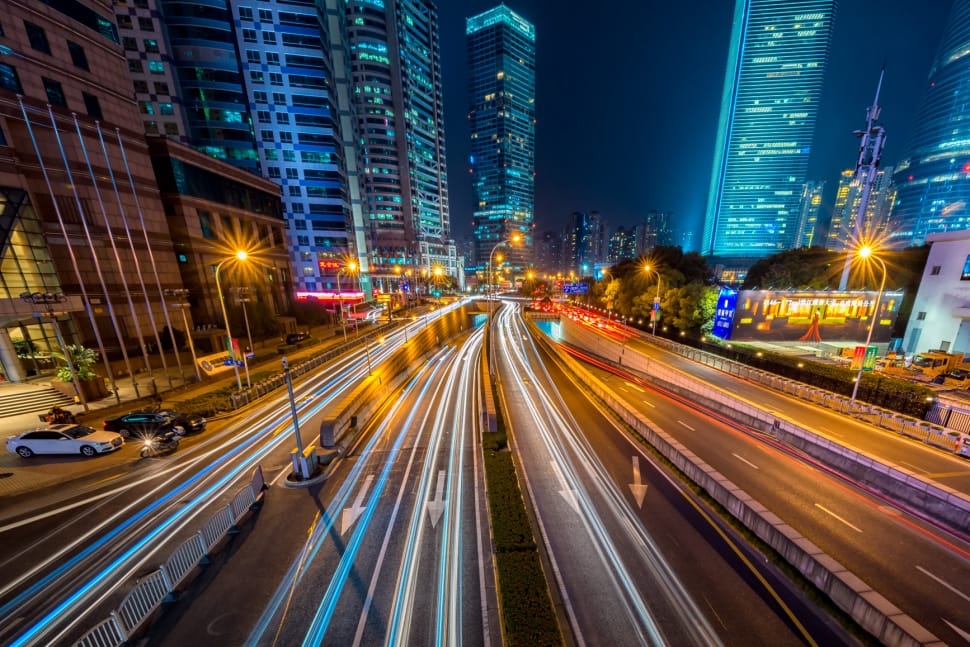
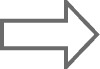
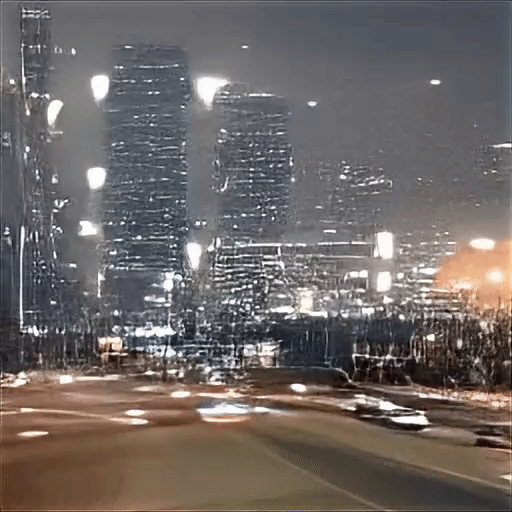
Image → Audio
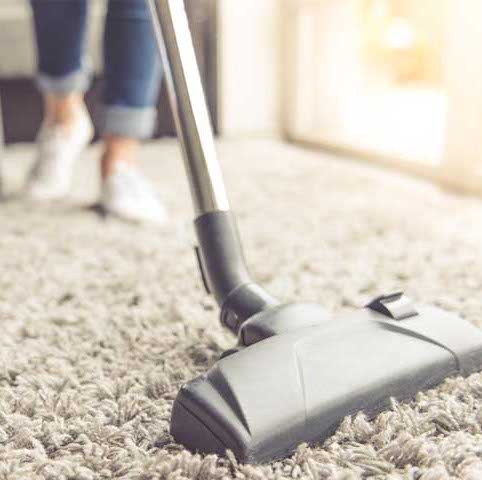
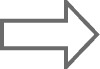
Audio → Text
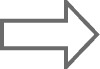
"A magical sound, game."
Image → Text
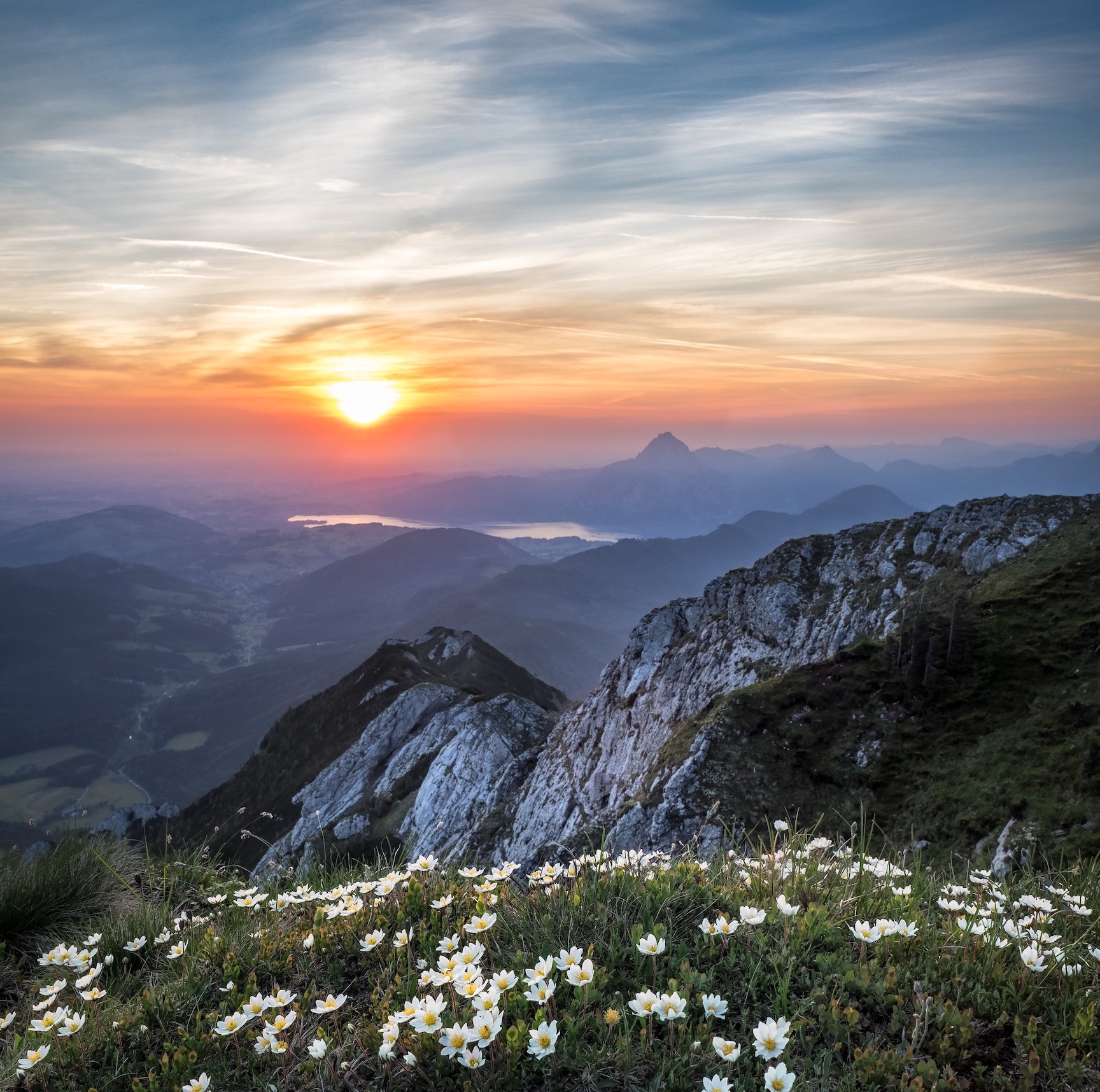
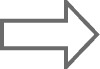
"Mountain view, sunset."
BibTeX
article{tang2023anytoany,
title={Any-to-Any Generation via Composable Diffusion},
author={Zineng Tang and Ziyi Yang and Chenguang Zhu and Michael Zeng and Mohit Bansal},
year={2023},
eprint={2305.11846},
archivePrefix={arXiv},
primaryClass={cs.CV}
}
标签:Diffusion,模态,via,Text,Image,CoDi,Video,Audio,Any
From: https://www.cnblogs.com/sddai/p/17545478.html