一、简介
Langchain-ChatGLM 相信大家都不陌生,近几周计划出一个源码解读,先解锁langchain的一些基础用法。
文档问答过程大概分为以下5部分,在Langchain中都有体现。
- 上传解析文档
- 文档向量化、存储
- 文档召回
- query向量化
- 文档问答
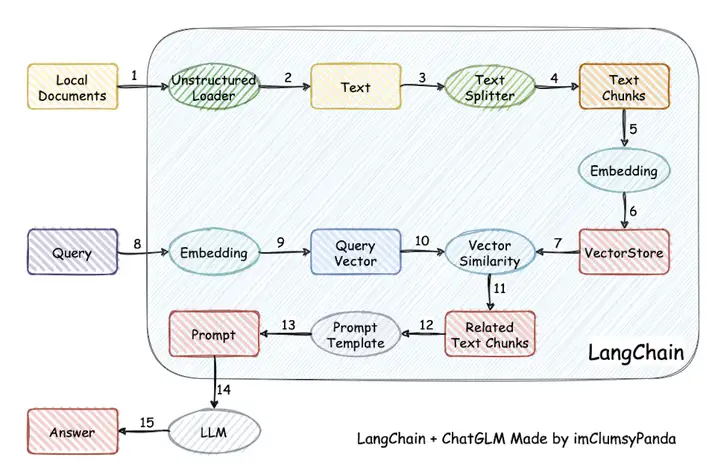
今天主要讲langchain在文档embedding以及构建faiss过程时是怎么实现的。
二、源码入口
langchain中对于文档embedding以及构建faiss过程有2个分支,
1.当第一次进行加载文件时如何生成faiss.index
2.当存在faiss.index时
下面也分别从这2个方面进行源码解读
if len(docs) > 0:
logger.info("文件加载完毕,正在生成向量库")
if vs_path and os.path.isdir(vs_path) and "index.faiss" in os.listdir(vs_path):
vector_store = load_vector_store(vs_path, self.embeddings)
vector_store.add_documents(docs)
torch_gc()
else:
if not vs_path:
vs_path = os.path.join(KB_ROOT_PATH,f"""{"".join(lazy_pinyin(os.path.splitext(file)[0]))}_FAISS_{datetime.datetime.now().strftime("%Y%m%d_%H%M%S")}""","vector_store")
vector_store = MyFAISS.from_documents(docs, self.embeddings) # docs 为Document列表
torch_gc()
vector_store.save_local(vs_path)
三、不存在faiss.index
MyFAISS.from_documents()重载了父类VectorStore的from_documents(),这里的self.embeddings其实是一个embedding对象
vector_store = MyFAISS.from_documents(docs, self.embeddings) # docs 为Document列表
self.embeddings = HuggingFaceEmbeddings(model_name=embedding_model_dict[embedding_model],
model_kwargs={'device': embedding_device})
@classmethod
def from_documents(
cls: Type[VST],
documents: List[Document],
embedding: Embeddings,
**kwargs: Any,) -> VST:
"""Return VectorStore initialized from documents and embeddings."""
texts = [d.page_content for d in documents]
metadatas = [d.metadata for d in documents]
return cls.from_texts(texts, embedding, metadatas=metadatas, **kwargs)
from_texts()主要做了3件事情
1.文档embedding化。
2. 创建内存中的文档存储
3.初始化FAISS数据库
最后返回的cls实例指class FAISS(VectorStore):
在这里,要注意2个变量,
embeddings: List[List[float]], 真正的embedding向量,2维列表
embedding: Embeddings, 一个huggingface类对象
@classmethod
def from_texts(
cls,
texts: List[str],
embedding: Embeddings,
metadatas: Optional[List[dict]] = None,
**kwargs: Any,) -> FAISS:
"""Construct FAISS wrapper from raw documents. This is a user friendly interface that: 1. Embeds documents. 2. Creates an in memory docstore 3. Initializes the FAISS database This is intended to be a quick way to get started. Example: .. code-block:: python from langchain import FAISS from langchain.embeddings import OpenAIEmbeddings embeddings = OpenAIEmbeddings() faiss = FAISS.from_texts(texts, embeddings) """
embeddings = embedding.embed_documents(texts)
return cls.__from(
texts,
embeddings,
embedding,
metadatas,
**kwargs, )
现在先看embeddings = embedding.embed_documents(texts)
我们在chains/modules/embeddings.py找到,可以看到embedding依赖client.encode这个函数,所以说如果想要自定义embedding模型,如果是huggingface的,那就比较简单,定义好模型名称和模型路径就可以了。这里返回一个向量List[List[float]]
def embed_documents(self, texts: List[str]) -> List[List[float]]:
"""Compute doc embeddings using a HuggingFace transformer model. Args: texts: The list of texts to embed. Returns: List of embeddings, one for each text. """
texts = list(map(lambda x: x.replace("\n", " "), texts))
embeddings = self.client.encode(texts, normalize_embeddings=True)
return embeddings.tolist()
self.client = sentence_transformers.SentenceTransformer(
self.model_name, cache_folder=self.cache_folder, **self.model_kwargs
)
接下来看cls.__from(),可以看出faiss构建索引的核心在这里面
@classmethod
def __from(
cls,
texts: List[str],
embeddings: List[List[float]],
embedding: Embeddings,
metadatas: Optional[List[dict]] = None,
normalize_L2: bool = False,
**kwargs: Any,) -> FAISS:
faiss = dependable_faiss_import()
index = faiss.IndexFlatL2(len(embeddings[0]))
vector = np.array(embeddings, dtype=np.float32)
if normalize_L2:
faiss.normalize_L2(vector)
index.add(vector)
documents = []
for i, text in enumerate(texts):
metadata = metadatas[i] if metadatas else {}
documents.append(Document(page_content=text, metadata=metadata))
index_to_id = {i: str(uuid.uuid4()) for i in range(len(documents))}
docstore = InMemoryDocstore(
{index_to_id[i]: doc for i, doc in enumerate(documents)}
)
return cls(
embedding.embed_query,
index,
docstore,
index_to_id,
normalize_L2=normalize_L2,
**kwargs, )
从中我们看到几个faiss构建索引的要素
1.faiss.IndexFlatL2,L2衡量距离
2.index_to_id ,给每个chunk一个独一无二的编码id,{hashcode:chunk1, ... ...}
3.docstore,其实是一个InMemoryDocstore类
class InMemoryDocstore(Docstore, AddableMixin):
"""Simple in memory docstore in the form of a dict."""
def __init__(self, _dict: Dict[str, Document]):
"""Initialize with dict."""
self._dict = _dict
def add(self, texts: Dict[str, Document]) -> None:
"""Add texts to in memory dictionary."""
overlapping = set(texts).intersection(self._dict)
if overlapping:
raise ValueError(f"Tried to add ids that already exist: {overlapping}")
self._dict = dict(self._dict, **texts)
def search(self, search: str) -> Union[str, Document]:
"""Search via direct lookup."""
if search not in self._dict:
return f"ID {search} not found."
else:
return self._dict[search]
4.embed_query(),这是HuggingFace的一个encoding的方法,这里真正实现了把文本进行向量化的过程
def embed_query(self, text: str) -> List[float]:
"""
Compute query embeddings using a HuggingFace transformer model.
Args:
text: The text to embed.
Returns: Embeddings for the text. """
text = text.replace("\n", " ")
embedding = self.client.encode(text, **self.encode_kwargs)
return embedding.tolist()
最后可以看到vector_store其实就是一个包含文档信息的FAISS对象,其中向量化的过程已经在流程中生成了文件
vector_store = MyFAISS.from_documents(docs, self.embeddings) # docs 为Document列表
class FAISS(VectorStore):
"""Wrapper around FAISS vector database. To use, you should have the ``faiss`` python package installed. Example: .. code-block:: python from langchain import FAISS faiss = FAISS(embedding_function, index, docstore, index_to_docstore_id) """
def __init__(
self,
embedding_function: Callable,
index: Any,
docstore: Docstore,
index_to_docstore_id: Dict[int, str],
relevance_score_fn: Optional[
Callable[[float], float]
] = _default_relevance_score_fn,
normalize_L2: bool = False, ):
三、存在faiss.index
vector_store = load_vector_store(vs_path, self.embeddings)
这里做了lru_cache缓存机制, MyFAISS调用静态方法load_local
@lru_cache(CACHED_VS_NUM)
def load_vector_store(vs_path, embeddings):
return MyFAISS.load_local(vs_path, embeddings)
可以看到最后返回的是一个vector_store的FAISS(VectorStore)类
@classmethoddef load_local(
cls, folder_path: str, embeddings: Embeddings, index_name: str = "index") -> FAISS:
"""Load FAISS index, docstore, and index_to_docstore_id to disk. Args: folder_path: folder path to load index, docstore, and index_to_docstore_id from. embeddings: Embeddings to use when generating queries index_name: for saving with a specific index file name """
path = Path(folder_path)
# load index separately since it is not picklable faiss = dependable_faiss_import()
index = faiss.read_index(
str(path / "{index_name}.faiss".format(index_name=index_name))
)
# load docstore and index_to_docstore_id with open(path / "{index_name}.pkl".format(index_name=index_name), "rb") as f:
docstore, index_to_docstore_id = pickle.load(f)
return cls(embeddings.embed_query, index, docstore, index_to_docstore_id)
进入主题,vector_store.add_documents(docs)嵌套了2个函数,依次如下
def add_documents(self, documents: List[Document], **kwargs: Any) -> List[str]:
"""Run more documents through the embeddings and add to the vectorstore. Args: documents (List[Document]: Documents to add to the vectorstore. Returns: List[str]: List of IDs of the added texts. """ # TODO: Handle the case where the user doesn't provide ids on the Collection texts = [doc.page_content for doc in documents]
metadatas = [doc.metadata for doc in documents]
return self.add_texts(texts, metadatas, **kwargs)
def add_texts(
self,
texts: Iterable[str],
metadatas: Optional[List[dict]] = None,
**kwargs: Any,) -> List[str]:
"""Run more texts through the embeddings and add to the vectorstore. Args: texts: Iterable of strings to add to the vectorstore. metadatas: Optional list of metadatas associated with the texts. Returns: List of ids from adding the texts into the vectorstore. """
if not isinstance(self.docstore, AddableMixin):
raise ValueError(
"If trying to add texts, the underlying docstore should support " f"adding items, which {self.docstore} does not" )
# Embed and create the documents. embeddings = [self.embedding_function(text) for text in texts]
return self.__add(texts, embeddings, metadatas, **kwargs)
def __add(
self,
texts: Iterable[str],
embeddings: Iterable[List[float]],
metadatas: Optional[List[dict]] = None,
**kwargs: Any,) -> List[str]:
if not isinstance(self.docstore, AddableMixin):
raise ValueError(
"If trying to add texts, the underlying docstore should support " f"adding items, which {self.docstore} does not" )
documents = []
for i, text in enumerate(texts):
metadata = metadatas[i] if metadatas else {}
documents.append(Document(page_content=text, metadata=metadata))
# Add to the index, the index_to_id mapping, and the docstore. starting_len = len(self.index_to_docstore_id)
faiss = dependable_faiss_import()
vector = np.array(embeddings, dtype=np.float32)
if self._normalize_L2:
faiss.normalize_L2(vector)
self.index.add(vector)
# Get list of index, id, and docs. full_info = [
(starting_len + i, str(uuid.uuid4()), doc)
for i, doc in enumerate(documents)
]
# Add information to docstore and index. self.docstore.add({_id: doc for _, _id, doc in full_info})
index_to_id = {index: _id for index, _id, _ in full_info}
self.index_to_docstore_id.update(index_to_id)
return [_id for _, _id, _ in full_info]
其中,self.index做的是向量增量操作;full_info,self.docstore,self.index_to_docstore_id做的都是数据增量操作,add_documents()返回如下,可以看出是一个文档编码list
full_info = [
(starting_len + i, str(uuid.uuid4()), doc)
for i, doc in enumerate(documents)
]
return [_id for _, _id, _ in full_info]
四、文件存储
文件存储,存了index、docstore、index_to_docstore_id,其中
{index_name}.faiss:向量存储的faiss索引
{index_name}.pkl:存取的docstore对象以及index_to_docstore_id字典
def save_local(self, folder_path: str, index_name: str = "index") -> None:
"""Save FAISS index, docstore, and index_to_docstore_id to disk. Args: folder_path: folder path to save index, docstore, and index_to_docstore_id to. index_name: for saving with a specific index file name """
path = Path(folder_path)
path.mkdir(exist_ok=True, parents=True)
# save index separately since it is not picklable faiss = dependable_faiss_import()
faiss.write_index(
self.index, str(path / "{index_name}.faiss".format(index_name=index_name))
)
# save docstore and index_to_docstore_id
with open(path / "{index_name}.pkl".format(index_name=index_name), "wb") as f:
pickle.dump((self.docstore, self.index_to_docstore_id), f)
五、杂谈
文档embedding以及构建faiss过程在实现中其实很绕,需要用心去读源码,还有关注函数define中的变量类型,理解才会事半功倍!
胖友,请不要忘了一键三连点赞哦!
转载请注明出处:QA Weekly
标签:index,embeddings,self,Langchain,id,texts,源码,embedding,docstore From: https://www.cnblogs.com/gongzb/p/18073884