转载:Python多进程运行——Multiprocessing基础教程2 - 知乎 (zhihu.com)
1 数据共享
在多进程处理中,所有新创建的进程都会有这两个特点:独立运行,有自己的内存空间。
我们来举个例子展示一下:
import multiprocessing
# empty list with global scope
result = []
def square_list(mylist):
global result
# append squares of mylist to global list result
for num in mylist:
result.append(num * num)
# print global list result
print("Result(in process p1): {}".format(result))
if __name__ == "__main__":
# input list
mylist = [1,2,3,4]
# creating new process
p1 = multiprocessing.Process(target=square_list, args=(mylist,))
# starting process
p1.start()
# wait until process is finished
p1.join()
# print global result list
print("Result(in main program): {}".format(result))
这个程序的输出结果是:
Result(in process p1): [1, 4, 9, 16]
Result(in main program): []
在上面的程序中我们尝试在两个地方打印全局列表result的内容:
- 在
square_list()
函数中,由于这个函数是由进程p1调用的,所以result列表只在进程p1的内存空间中更改。 - 在主程序中的p1进程完成后。由于主程序由不同的进程运行,它的内存空间中的result列表仍然是空的。
我们再用一张图来帮助理解记忆不同进程间的数据关系:
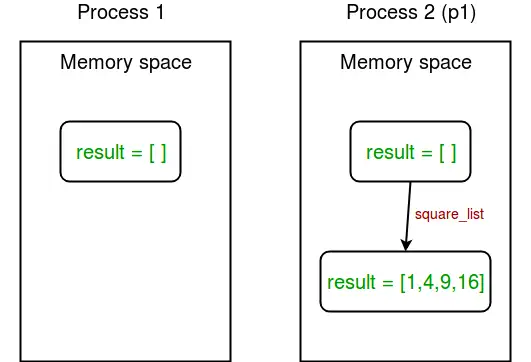
图1 进程间数据关系
1.1 内存共享
如果程序需要在不同的进程之间共享一些数据的话,该怎么做呢?不用担心,multiprocessing模块提供了Array对象和Value对象,用来在进程之间共享数据。
所谓Array对象和Value对象分别是指从共享内存中分配的ctypes数组和对象。我们直接来看一个例子,展示如何用Array对象和Value对象在进程之间共享数据:
import multiprocessing
def square_list(mylist, result, square_sum):
# append squares of mylist to result array
for idx, num in enumerate(mylist):
result[idx] = num * num
# square_sum value
square_sum.value = sum(result)
# print result Array
print("Result(in process p1): {}".format(result[:]))
# print square_sum Value
print("Sum of squares(in process p1): {}".format(square_sum.value))
if __name__ == "__main__":
# input list
mylist = [1,2,3,4]
# creating Array of int data type with space for 4 integers
result = multiprocessing.Array('i', 4)
# creating Value of int data type
square_sum = multiprocessing.Value('i')
# creating new process
p1 = multiprocessing.Process(target=square_list, args=(mylist, result, square_sum))
# starting process
p1.start()
# wait until process is finished
p1.join()
# print result array
print("Result(in main program): {}".format(result[:]))
# print square_sum Value
print("Sum of squares(in main program): {}".format(square_sum.value))
程序输出的结果如下:
Result(in process p1): [1, 4, 9, 16]
Sum of squares(in process p1): 30
Result(in main program): [1, 4, 9, 16]
Sum of squares(in main program): 30
成功了!主程序和p1进程输出了同样的结果,说明程序中确实完成了不同进程间的数据共享。那么我们来详细看一下上面的程序做了什么:
在主程序中我们首先创建了一个Array对象:
result = multiprocessing.Array('i', 4)
向这个对象输入的第一个参数是数据类型:i表示整数,d代表浮点数。第二个参数是数组的大小,在这个例子中我们创建了包含4个元素的数组。
类似的,我们创建了一个Value对象:
square_sum = multiprocessing.Value('i')
我们只对Value对象输入了一个参数,那就是数据类型,与上述的方法一致。当然,我们还可以对其指定一个初始值(比如10),就像这样:
square_sum = multiprocessing.Value('i', 10)
随后,我们在创建进程对象时,将刚创建好的两个对象:result和square_sum作为参数输入给进程:
p1 = multiprocessing.Process(target=square_list, args=(mylist, result, square_sum))
在函数中result元素通过索引进行数组赋值,square_sum通过value
属性进行赋值。
注意:为了完整打印result数组的结果,需要使用result[:]
进行打印,而square_sum也需要使用value
属性进行打印:
print("Result(in process p1): {}".format(result[:]))
print("Sum of squares(in process p1): {}".format(square_sum.value))
1.2 服务器进程
每当python程序启动时,同时也会启动一个服务器进程。随后,只要我们需要生成一个新进程,父进程就会连接到服务器并请求它派生一个新进程。这个服务器进程可以保存Python对象,并允许其他进程使用代理来操作它们。
multiprocessing模块提供了能够控制服务器进程的Manager类。所以,Manager类也提供了一种创建可以在不同流程之间共享的数据的方法。
服务器进程管理器比使用共享内存对象更灵活,因为它们可以支持任意对象类型,如列表、字典、队列、值、数组等。此外,单个管理器可以由网络上不同计算机上的进程共享。
但是,服务器进程管理器的速度比使用共享内存要慢。
让我们来看一个例子:
import multiprocessing
def print_records(records):
for record in records:
print("Name: {0}\nScore: {1}\n".format(record[0], record[1]))
def insert_record(record, records):
records.append(record)
print("New record added!\n")
if __name__ == '__main__':
with multiprocessing.Manager() as manager:
# creating a list in server process memory
records = manager.list([('Sam', 10), ('Adam', 9), ('Kevin',9)])
# new record to be inserted in records
new_record = ('Jeff', 8)
# creating new processes
p1 = multiprocessing.Process(target=insert_record, args=(new_record, records))
p2 = multiprocessing.Process(target=print_records, args=(records,))
# running process p1 to insert new record
p1.start()
p1.join()
# running process p2 to print records
p2.start()
p2.join()
这个程序的输出结果是:
New record added!
Name: Sam
Score: 10
Name: Adam
Score: 9
Name: Kevin
Score: 9
Name: Jeff
Score: 8
我们来理解一下这个程序做了什么:首先我们创建了一个manager对象
with multiprocessing.Manager() as manager:
在with语句下的所有行,都是在manager对象的范围内的。接下来我们使用这个manager对象创建了列表(类似的,我们还可以用manager.dict()
创建字典)。
最后我们创建了进程p1(用于在records列表中插入一条新的record)和p2(将records打印出来),并将records作为参数进行传递。
服务器进程的概念再次用下图总结一下:
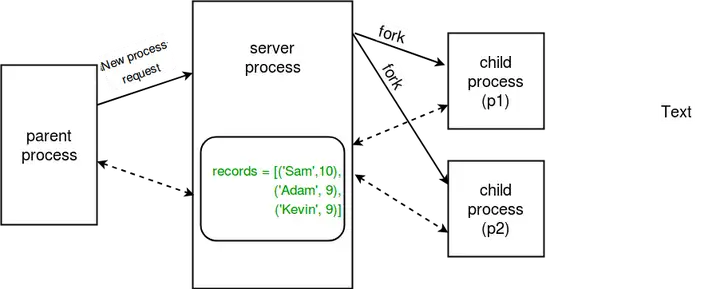
图2 服务器进程数据共享
2 数据传递
为了能使多个流程能够正常工作,常常需要在它们之间进行一些通信,以便能够划分工作并汇总最后的结果。multiprocessing模块支持进程之间的两种通信通道:Queue和Pipe。
2.1 Queue
使用队列来回处理多进程之间的通信是一种比较简单的方法。任何Python对象都可以使用队列进行传递。我们来看一个例子:
import multiprocessing
def square_list(mylist, q):
# append squares of mylist to queue
for num in mylist:
q.put(num * num)
def print_queue(q):
print("Queue elements:")
while not q.empty():
print(q.get())
print("Queue is now empty!")
if __name__ == "__main__":
# input list
mylist = [1,2,3,4]
# creating multiprocessing Queue
q = multiprocessing.Queue()
# creating new processes
p1 = multiprocessing.Process(target=square_list, args=(mylist, q))
p2 = multiprocessing.Process(target=print_queue, args=(q,))
# running process p1 to square list
p1.start()
p1.join()
# running process p2 to get queue elements
p2.start()
p2.join()
上面这个程序的输出结果是:
Queue elements:
1
4
9
16
Queue is now empty!
我们来看一下上面这个程序到底做了什么。首先我们创建了一个Queue对象:
q = multiprocessing.Queue()
然后,将这个空的Queue对象输入square_list函数。该函数会将列表中的数平方,再使用put()
方法放入队列中:
q.put(num * num)
随后使用get()
方法,将q打印出来,直至q重新称为一个空的Queue对象:
while not q.empty():
print(q.get())
我们还是用一张图来帮助理解记忆:
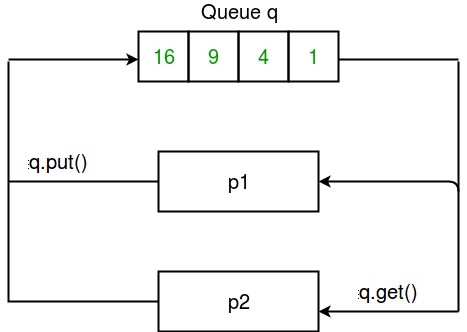
图2 服务器进程数据共享
2.2 Pipe
一个Pipe对象只能有两个端点。因此,当进程只需要双向通信时,它会比Queue对象更好用。
multiprocessing模块提供了Pipe()
函数,该函数返回由管道连接的一对连接对象。Pipe()
返回的两个连接对象分别表示管道的两端。每个连接对象都有send()
和recv()
方法。
我们来看一个例子:
import multiprocessing
def sender(conn, msgs):
for msg in msgs:
conn.send(msg)
print("Sent the message: {}".format(msg))
conn.close()
def receiver(conn):
while 1:
msg = conn.recv()
if msg == "END":
break
print("Received the message: {}".format(msg))
if __name__ == "__main__":
# messages to be sent
msgs = ["hello", "hey", "hru?", "END"]
# creating a pipe
parent_conn, child_conn = multiprocessing.Pipe()
# creating new processes
p1 = multiprocessing.Process(target=sender, args=(parent_conn,msgs))
p2 = multiprocessing.Process(target=receiver, args=(child_conn,))
# running processes
p1.start()
p2.start()
# wait until processes finish
p1.join()
p2.join()
上面这个程序的输出结果是:
Sent the message: hello
Sent the message: hey
Sent the message: hru?
Received the message: hello
Sent the message: END
Received the message: hey
Received the message: hru?
我们还是来看一下这个程序到底做了什么。首先创建了一个Pipe对象:
parent_conn, child_conn = multiprocessing.Pipe()
与上文说的一样,该对象返回了一对管道两端的两个连接对象。然后使用send()
方法和recv()
方法进行信息的传递。就这么简单。在上面的程序中,我们从一端向另一端发送一串消息。在另一端,我们收到消息,并在收到END消息时退出。
要注意的是,如果两个进程(或线程)同时尝试从管道的同一端读取或写入管道中的数据,则管道中的数据可能会损坏。不过不同的进程同时使用管道的两端是没有问题的。还要注意,Queue对象在进程之间进行了适当的同步,但代价是增加了计算复杂度。因此,Queue对象对于线程和进程是相对安全的。
最后我们还是用一张图来示意:
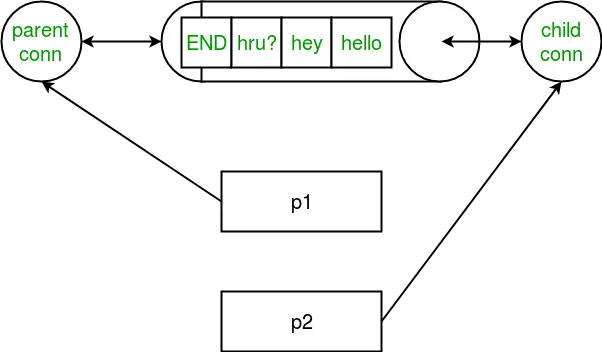
图4 用Pipe完成进程间数据传输
标签:p1,Python,multiprocessing,进程,square,result,基础教程,print,Multiprocessing From: https://www.cnblogs.com/zhiminyu/p/17421123.html